Digital Twins: A Comprehensive Guide for 2024
By bridging the physical and digital worlds, digital twin technology has significantly transformed the way various industries operate. They are useful for a wide range of professionals, including engineers, architects, designers, healthcare professionals, and even real estate marketers.
_YouTube_Thumbnail_1280x720_px_(15).jpg)
A digital twin, also known as a virtual twin, is a digital model designed to accurately reflect a physical object, system, process, place, or even a person, and are used to gain insights into the present and future states of the physical twin.
By bridging the physical and digital worlds, digital twin technology has significantly transformed the way various industries operate. They are useful for a wide range of professionals, including engineers, architects, designers, healthcare professionals, and even real estate marketers.
If you've already recognized the potential value of digital twins and are eager to leverage this innovative technology for streamlining processes, improving decision-making, and fostering innovation, this comprehensive guide will guide you toward a successful implementation.
You can expect us to cover the following topics:
- History and Evolution of Digital Twin Technology
- Key Components of a Digital Twin
- Benefits of Digital Twins
- Challenges and Limitations of Digital Twins
- How to Create a Digital Twin
- Integrating Digital Twins with AR/VR
- Data Management Strategies
- The Importance of Standardization and Interoperability
- Cybersecurity Considerations
- Case Studies
- The Future Outlook of Digital Twin Technology
- A Recap of Key Takeaways and Steps Forward
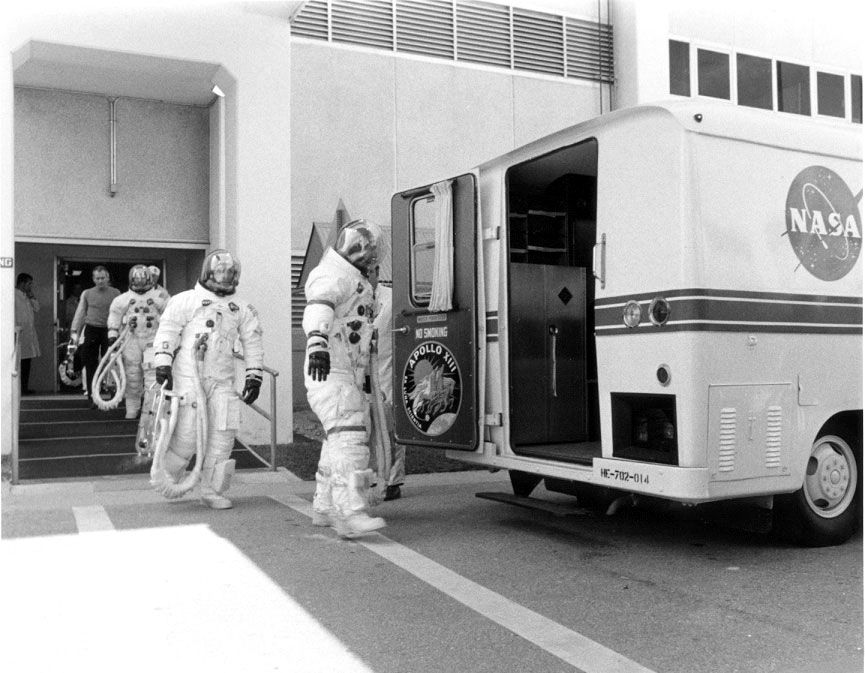
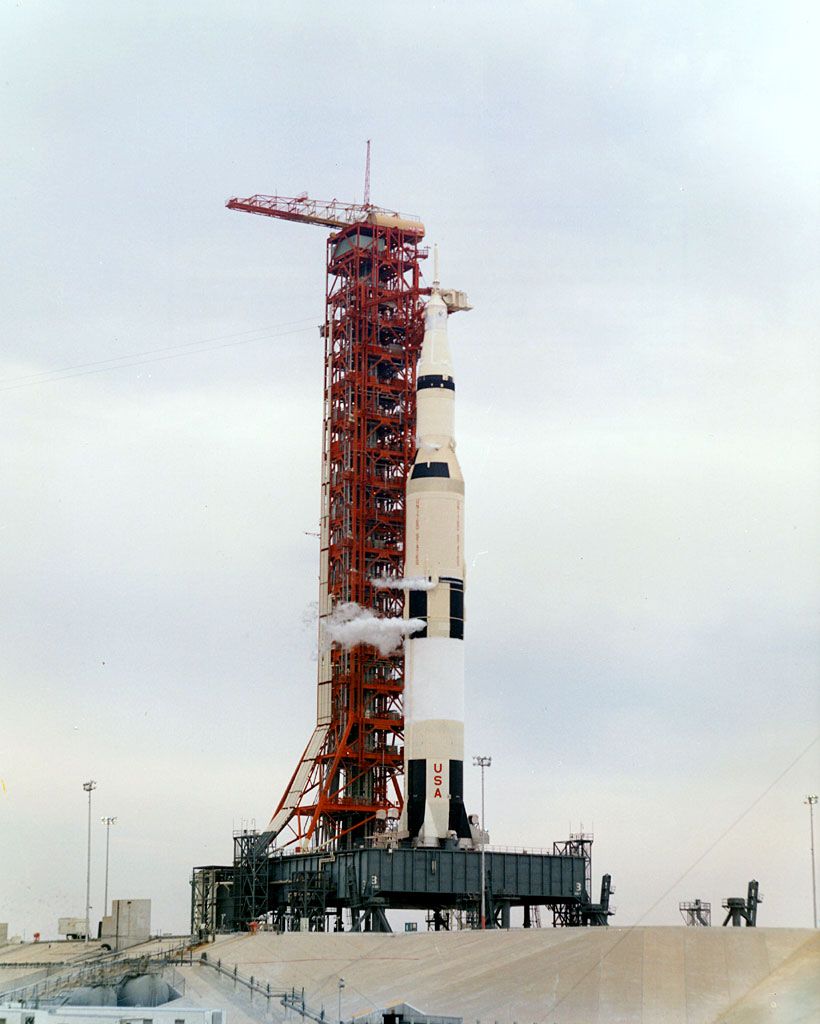
History and Evolution of Digital Twin Technology
Understanding their origin of digital twins provides valuable insights into their current capabilities and future potential. The concept, once a theoretical ideal, has evolved into a cornerstone of modern industry and technology. This section covers the progressive stages and significant milestones that have shaped the development of digital twin technology.
Key Milestones
- Conceptualization (1970s): After the oxygen tank explosion on Apollo 13 damaged the main engine, NASA used various simulators to understand the failure. They then expanded a physical model of the spacecraft with digital elements, creating the first-ever digital twin. This digital twin continuously collected data, helping experts analyze what led to the accident and plan future actions.
- Experimentation and Development (1990s): In the 1990s, NASA started experimenting with digital twin concepts to create virtual replicas of spacecraft and other space exploration assets like the Mars rovers (Spirit and Opportunity). This enabled engineers to simulate and plan maneuvers on the Martian surface before execution.
- Formally Defined (2002): Dr. Michael Grieves (current Executive Director of the Digital Twin Institute and former NASA consultant) defines the digital twin as consisting of three parts: real space, virtual space, and the link for data flow from real space to virtual space. (You can read more about his work here.)
- Advancement in Sensor Technologies (Mid-2000s): In the mid-2000s, advancements in sensor technologies and data collection methods began to facilitate the integration of relevant data from physical entities into digital twin environments.
- Real-Time Data Development (Late 2000s): During the late 2000s, the integration of real-time data became more sophisticated, ensuring that digital twins could dynamically reflect the current state of their physical counterparts.
- Simulation and Analysis Tools Integration (2010s): In the 2010s, advancements in simulation and analysis tools were integrated into digital twin environments, enhancing the capability to virtually simulate scenarios and optimize processes.
- Interdisciplinary Collaboration (2010s): As digital twin technology expanded, the trend of collaboration among professionals with diverse expertise became increasingly prevalent. This collaborative approach fostered an environment where experts from various fields worked together, leveraging their respective skills to harness the full potential of digital twins.
- Predictive Analytics Implementation (2010s): In the 2010s, the implementation of predictive analytics algorithms became more prevalent, allowing digital twins to anticipate future states and potential issues based on historical and real-time data.
- User Interface and Accessibility (2010s): Throughout the 2010s, efforts were made to improve user interfaces and accessibility, making it easier for professionals from different fields to interact with and extract insights from digital twins.
- Integration with AI (Ongoing): Digital twins are becoming more integrated with AI, improving its ability to predict future performance, optimize operations, and drive innovation across an array of fields.
These dates provide a general overview, and it's important to note that digital twin technology is always changing, with new advancements continuously influencing its direction.
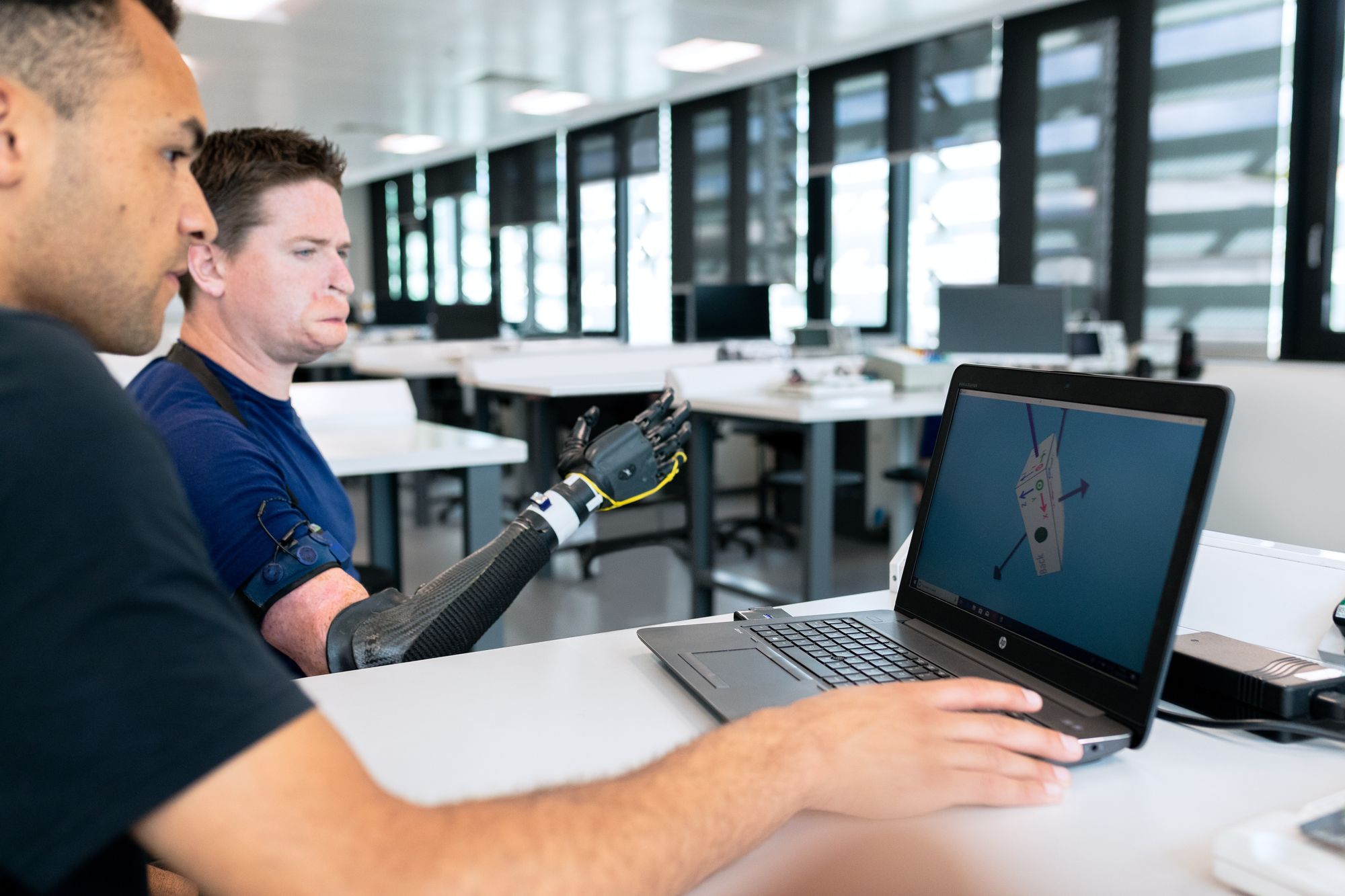
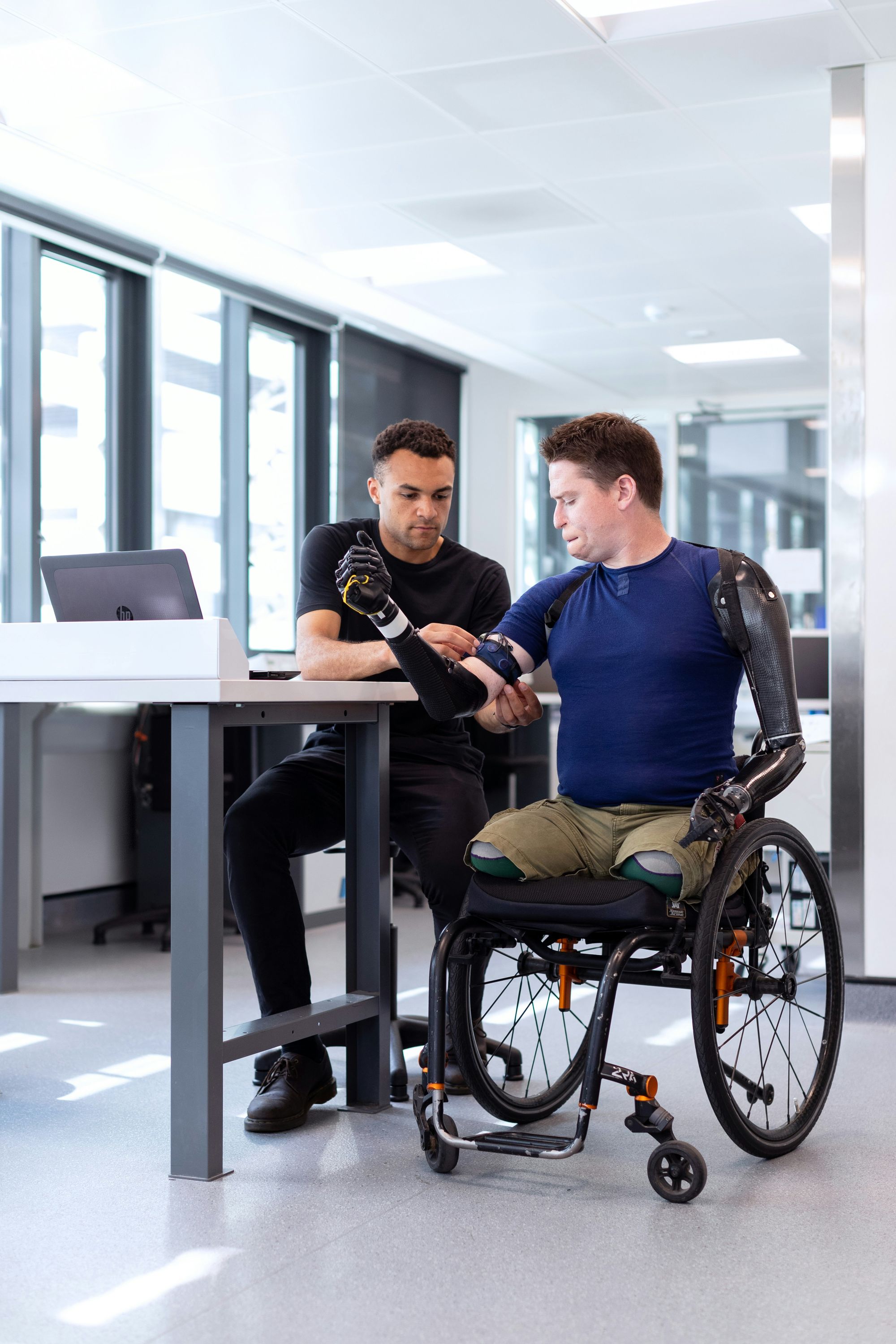
Key Components of a Digital Twin
Digital twins are composed of the following three elements:
- real entity
- virtual representation
- data link that connects the two (digital thread)
.jpg)
Beyond these fundamental elements, digital twins may also have the following components that play a role in ensuring the digital twin accurately reflects its physical counterpart.
Sensors
Sensors serve as the sensory organs of digital twins, capturing real-time data related to performance, environment, and utilization. This network of interconnected sensors ensures the digital twin offers a live reflection of the physical object, allowing for prompt maintenance, fine-tuning, and the anticipation of potential issues before they arise.
Software
Software is critical to the operation of a digital twin. It involves the synchronization of various applications and modeling tools that power the digital twin, allowing it to simulate, predict, and optimize the performance of its physical counterpart. This integration enables intricate virtual representations that can evolve, learn, and provide value across multiple stages of the asset's lifespan.
Internet of Things (IoT)
The Internet of Things (IoT) is fundamental to digital twin technology. IoT devices act as sensory input mechanisms, gathering real-time data from various sources, including machines, environmental sensors, and even entire factories. This network of connected devices provides a continuous stream of information that feeds digital twins, allowing them to mirror the physical world accurately.
AI and ML
Intelligence is the hallmark of any advanced system. Artificial Intelligence (AI) and Machine Learning (ML) imbue digital twins with the capability to analyze data, make predictions, and learn from outcomes to enhance performance. AI algorithms can detect patterns and anomalies, while ML models evolve through iterative training, improving the digital twin's predictive accuracy and operational efficiency.
Together, these components form the robust architecture of a digital twin, allowing for a versatile and powerful tool that drives innovation and efficiency in various industries.
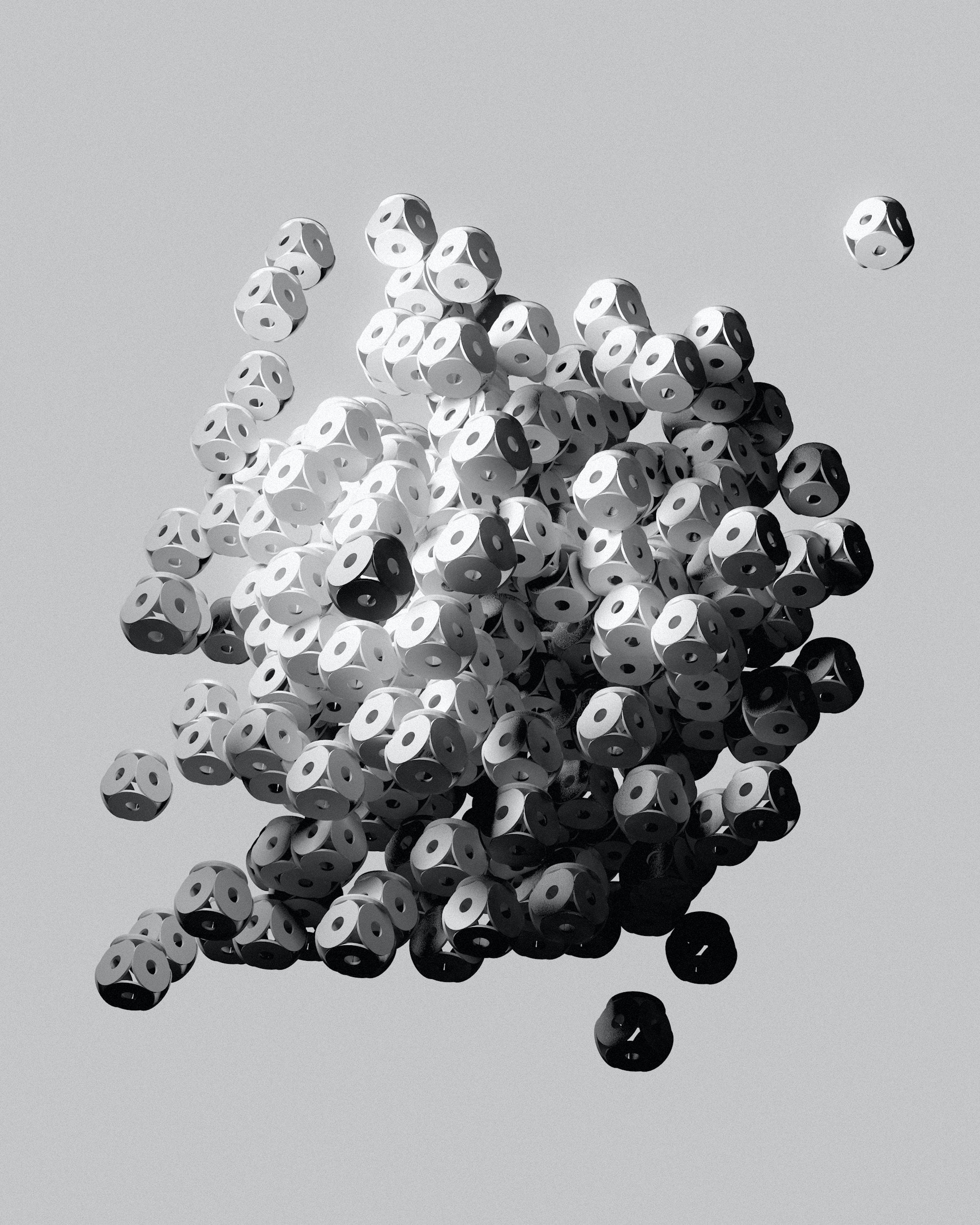
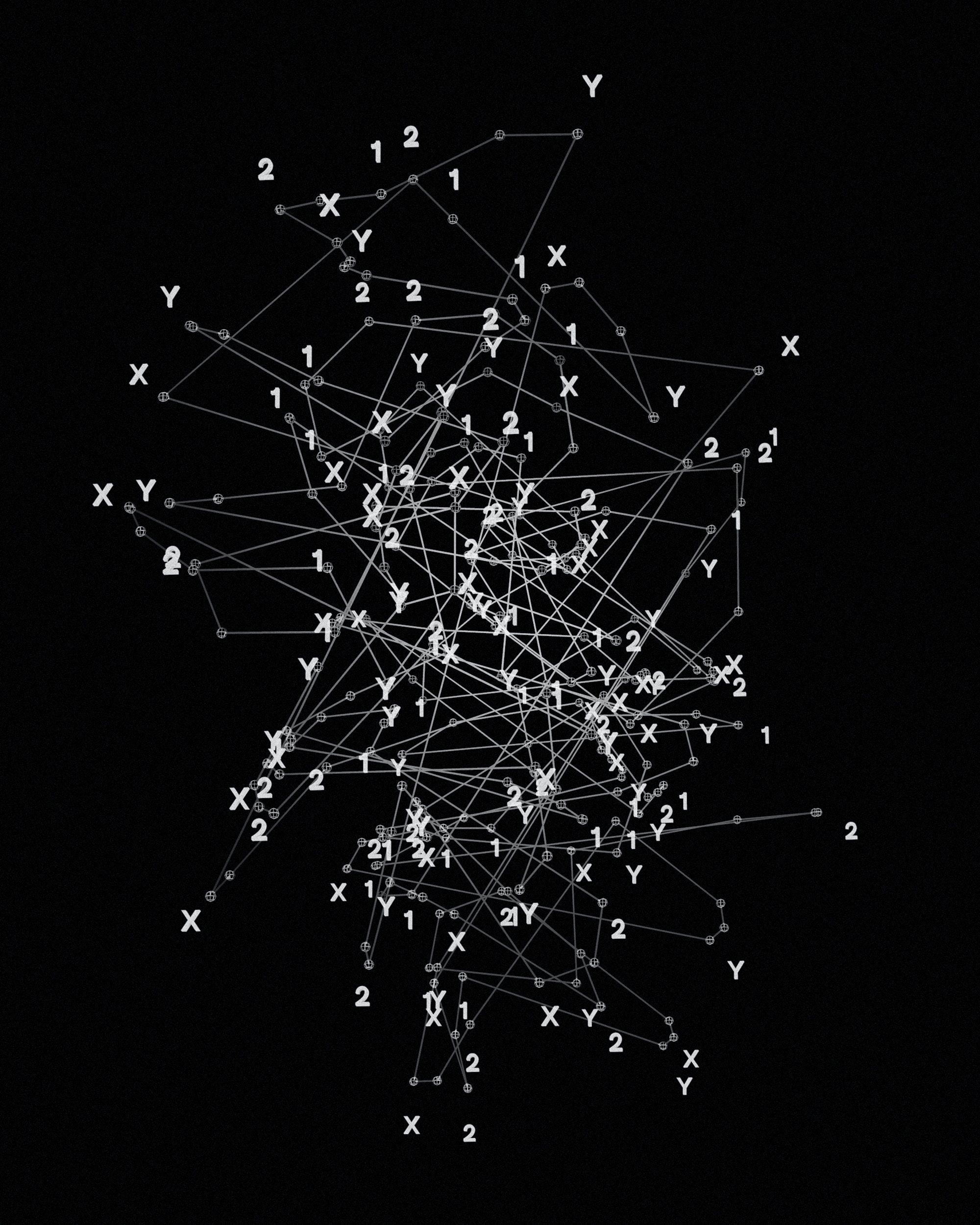
Benefits of Digital Twins
1. Improve Efficiency and Reduce Costs
Digital Twins play a critical role in optimizing operations, leading to enhanced efficiency and substantial cost reductions. By creating a virtual representation of a physical system or process, organizations can also identify design flaws and test solutions in a virtual environment, thereby saving time and resources.
2. Mitigate Risk
Digital twins enable companies to foresee potential issues before they escalate by simulating scenarios and monitoring the real-time data of their physical counterparts. This predictive capacity facilitates timely maintenance, helping to avoid downtime and reduce repair costs.
3. Accelerate Innovation
Digital twin technology accelerates innovation by allowing businesses to iterate designs and test products before they are built. It radically improves product lifecycle management by offering insights into each stage of a product's life, from conception to disposal.
4. Predict Trends and Logistical Scenarios
Advanced analytics and machine learning algorithms embedded in digital twins analyze historical patterns and current data to predict future trends. For instance, they can forecast demand patterns, identify potential bottlenecks, or predict the impact of disruptions on the supply chain.
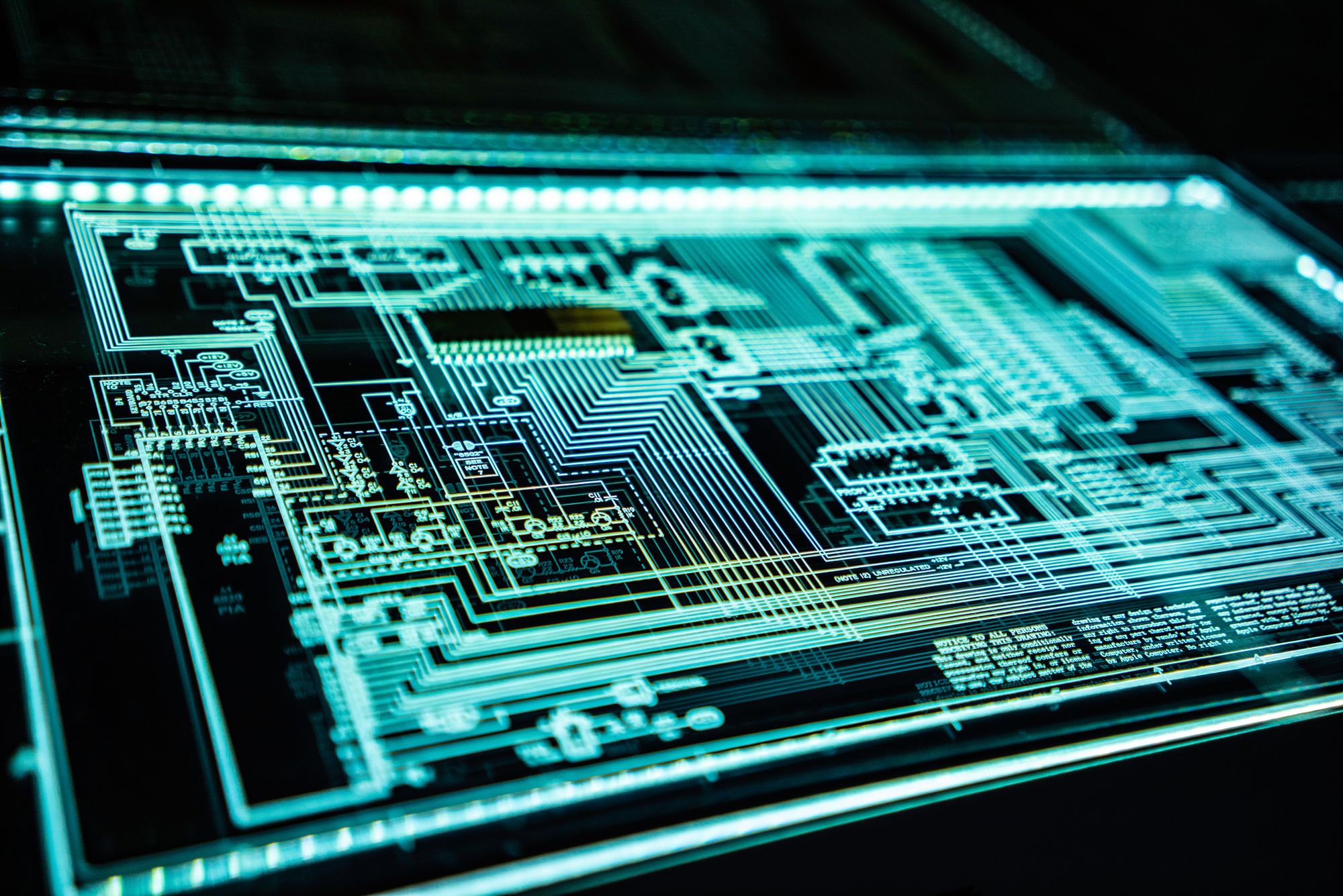
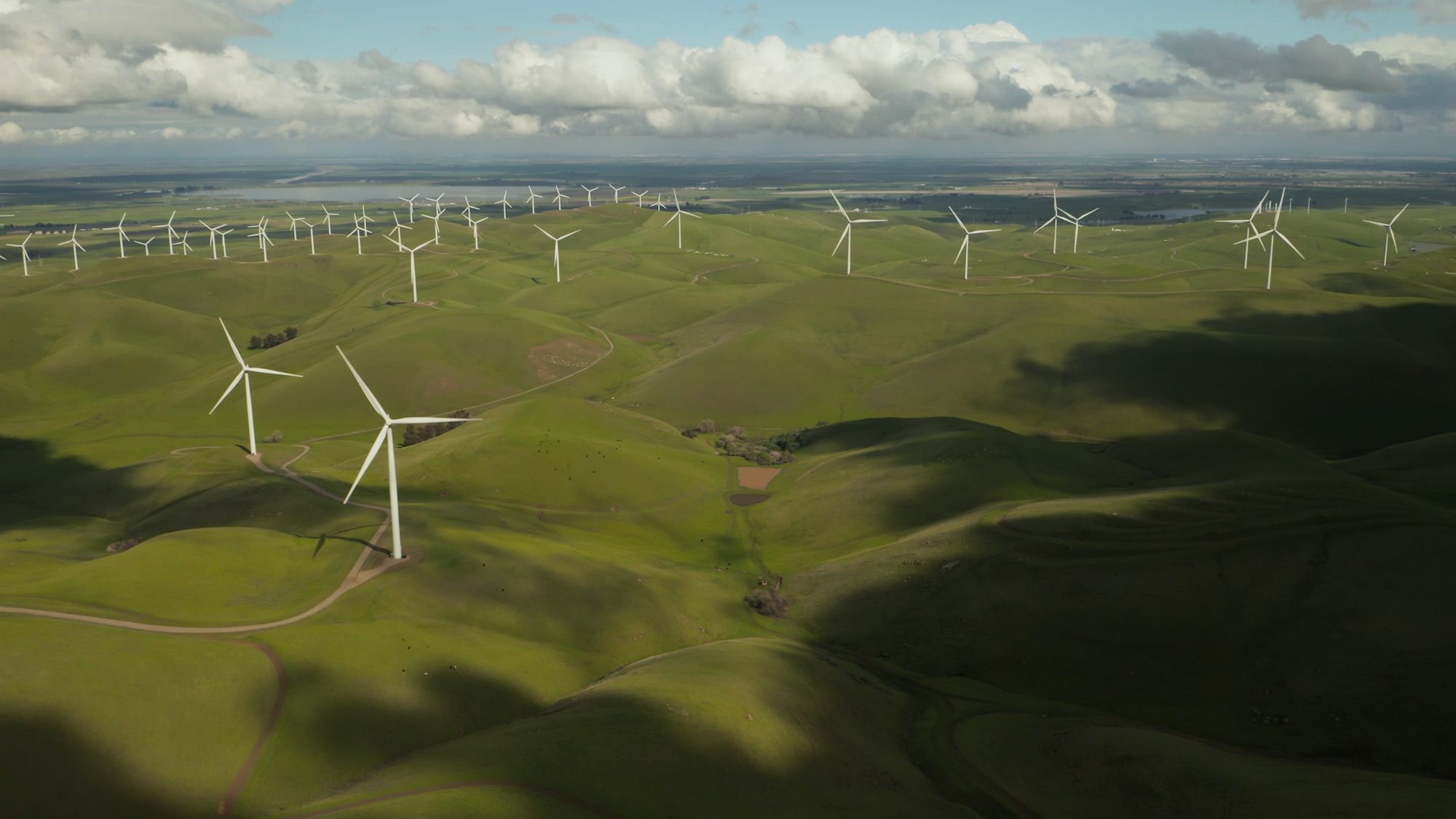
Challenges and Limitations of Digital Twins
As with any transformative technology, the implementation and operation of digital twins come with their own set of challenges and limitations. Businesses and organizations looking to leverage digital twins must be aware of these hurdles to effectively plan and execute their digital twin strategies.
1. Addressing Technical and Operational Issues
The technical complexities of creating a digital twin are non-trivial. Ensuring accurate real-time data synchronization, integrating disparate systems, and maintaining high-performance computing environments demand a robust IT infrastructure. Operational challenges include the need for specialized expertise to design, develop, and manage digital twins, as well as issues related to maintenance.
2. Bridging the Gap Between Expectations and Reality
It is vital to set realistic expectations when deploying digital twins. The promise of comprehensive optimization and predictive analytics may fall short due to data quality issues, incomplete models, or unexpected variables in the physical system. Bridging the gap between expectations and reality requires a clear understanding of the technology's capabilities and limitations.
3. Navigating the Complexity of Implementation
Implementing a digital twin can be a complex process involving multiple stakeholders and systems. The challenges include not only the technical aspects but also organizational hurdles such as resistance to change, misalignment between departments, and ensuring regulatory compliance. Developing a streamlined implementation strategy is crucial to minimize disruptions and realize the full benefits of digital twins.
- Technical Integration: Seamlessly integrating digital twins with existing systems can be difficult, as it often requires bespoke solutions and continuous adaptation.
- Data Privacy and Security: Protecting sensitive information and ensuring robust cybersecurity measures in a digital twin environment is a significant concern, given the potential for data breaches.
- Interoperability: Digital twins must be able to communicate with other systems and technologies, which requires a set of shared standards and protocols that may not be fully developed or adopted industry-wide.
- Cost: The initial investment for developing and implementing a digital twin can be high, making it necessary to carefully evaluate the return on investment and long-term benefits.
4. Conquering Scale-Up Struggles
Coordinating and synchronizing multiple digital twins within a larger system pose complexities in data orchestration and ensuring seamless interoperability. This underscores the importance of addressing scalability challenges not only at the individual digital twin level but also in the context of a comprehensive and interconnected system.
It is important to set realistic expectations when deploying digital twins within a larger framework to maximize their effectiveness across complex organizational and technological landscapes.
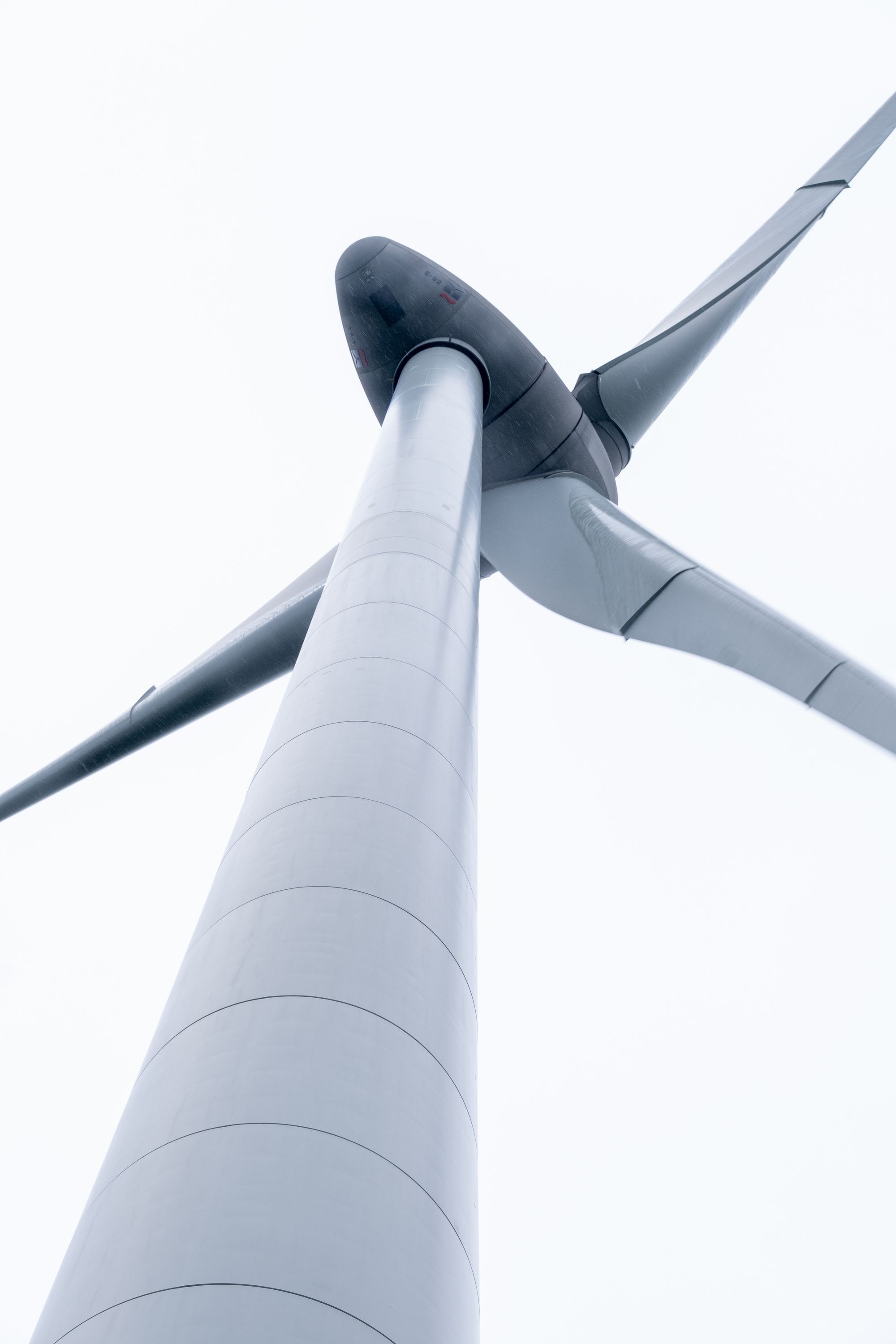
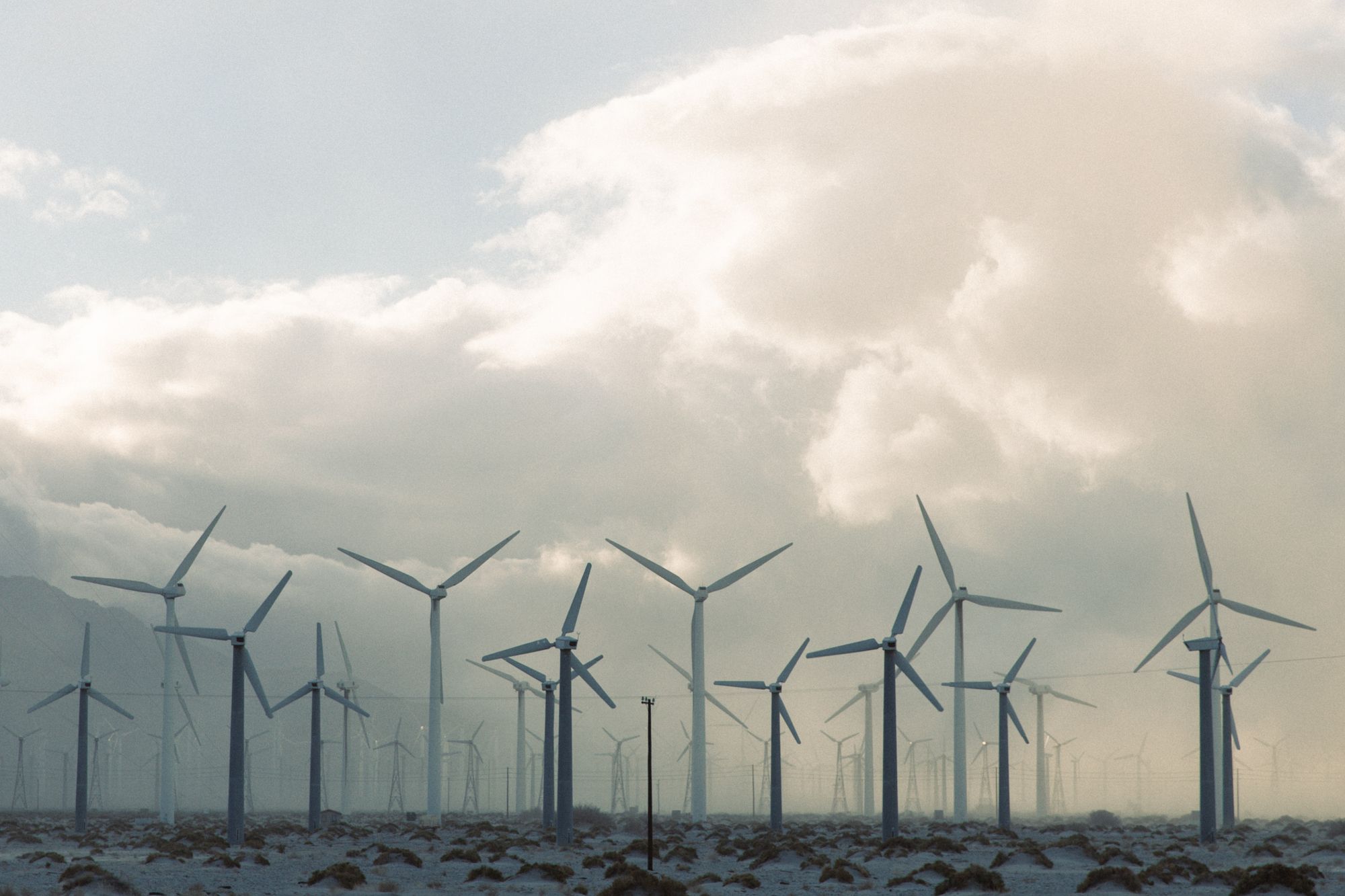
How to Create a Digital Twin
While methods will vary based on the use case, this segment provides a general roadmap for those looking to create a digital twin.
Phase 1: Assessing Organizational Readiness
Before you can begin implementing digital twin technology, it is vital to evaluate your organization's current state.
Consider:
- Are your systems compatible with a digital twin platform?
- Is your data structured in a way that can be mirrored virtually
- How mature is your existing infrastructure? This may include hardware and software assets, data management, and process integration.
Phase 2: Build a Skilled Team to Manage Twin Development and Monitoring
Incorporating digital twins into your business processes demands a blend of cross-disciplinary skills. Look to recruit or train professionals who can navigate the complex interplay of physical and virtual systems.
We recommend assembling a team with expertise in areas such as:
- IoT
- AI
- Data analytics
- Domain-specific knowledge
Phase 3: Develop a Roadmap for Integration and Scalability
A structured roadmap is key when it comes to integrating digital twins into your organizational ecosystem. Your roadmap will rely on the expertise and capacity of your team.
We recommend:
- Starting with small-scale pilot projects which can provide valuable insights and pave the way for broader functional applications
- Creating a phased approach to minimize disruption
- Define key milestones and deliverables to ensure progress
- Identify pilot projects to validate concepts and gauge ROI
- Decide on benchmarks to measure the success of your digital twin implementations
- Plan for scalability to adapt to evolving business needs and technological advancements
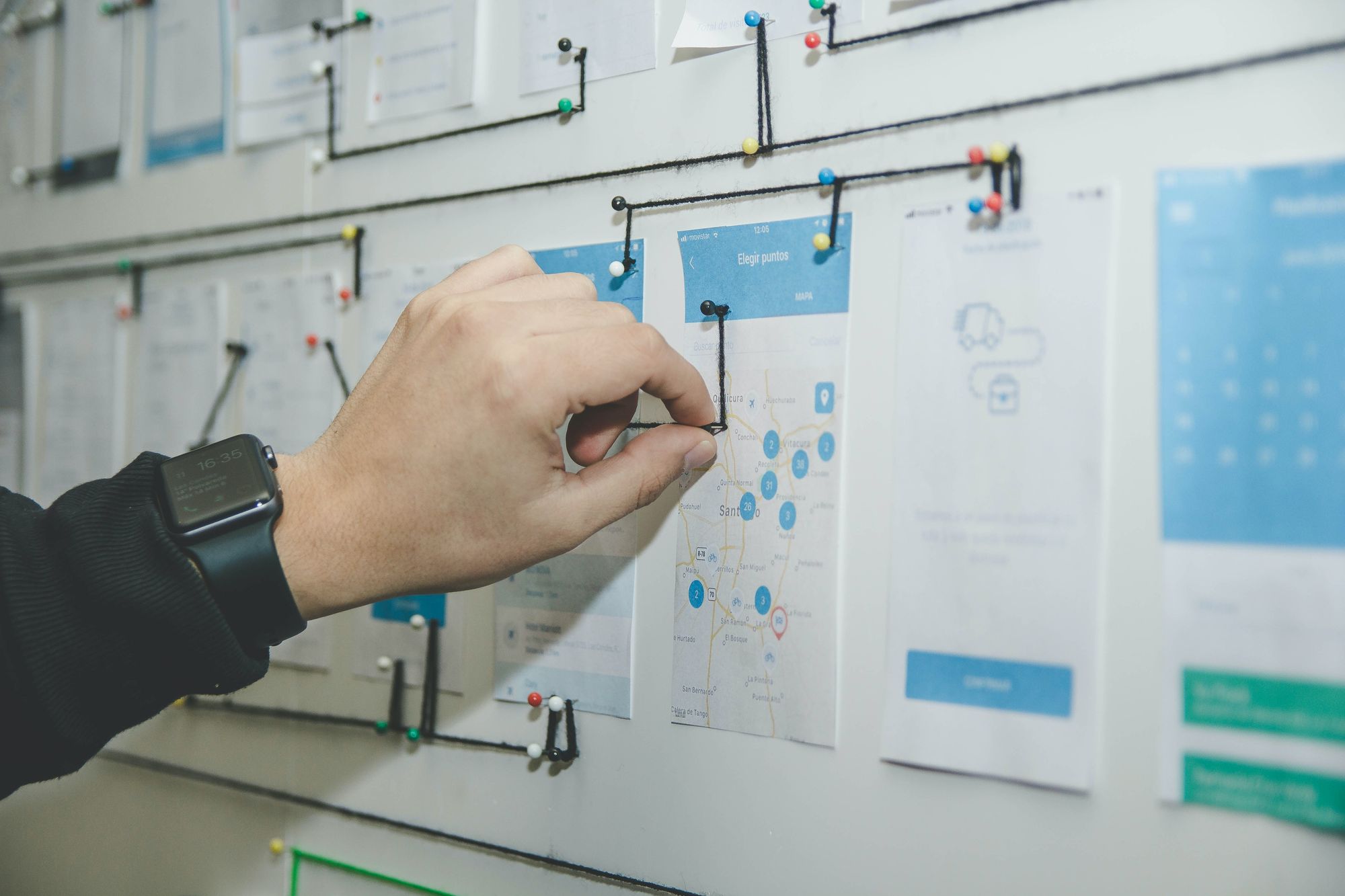
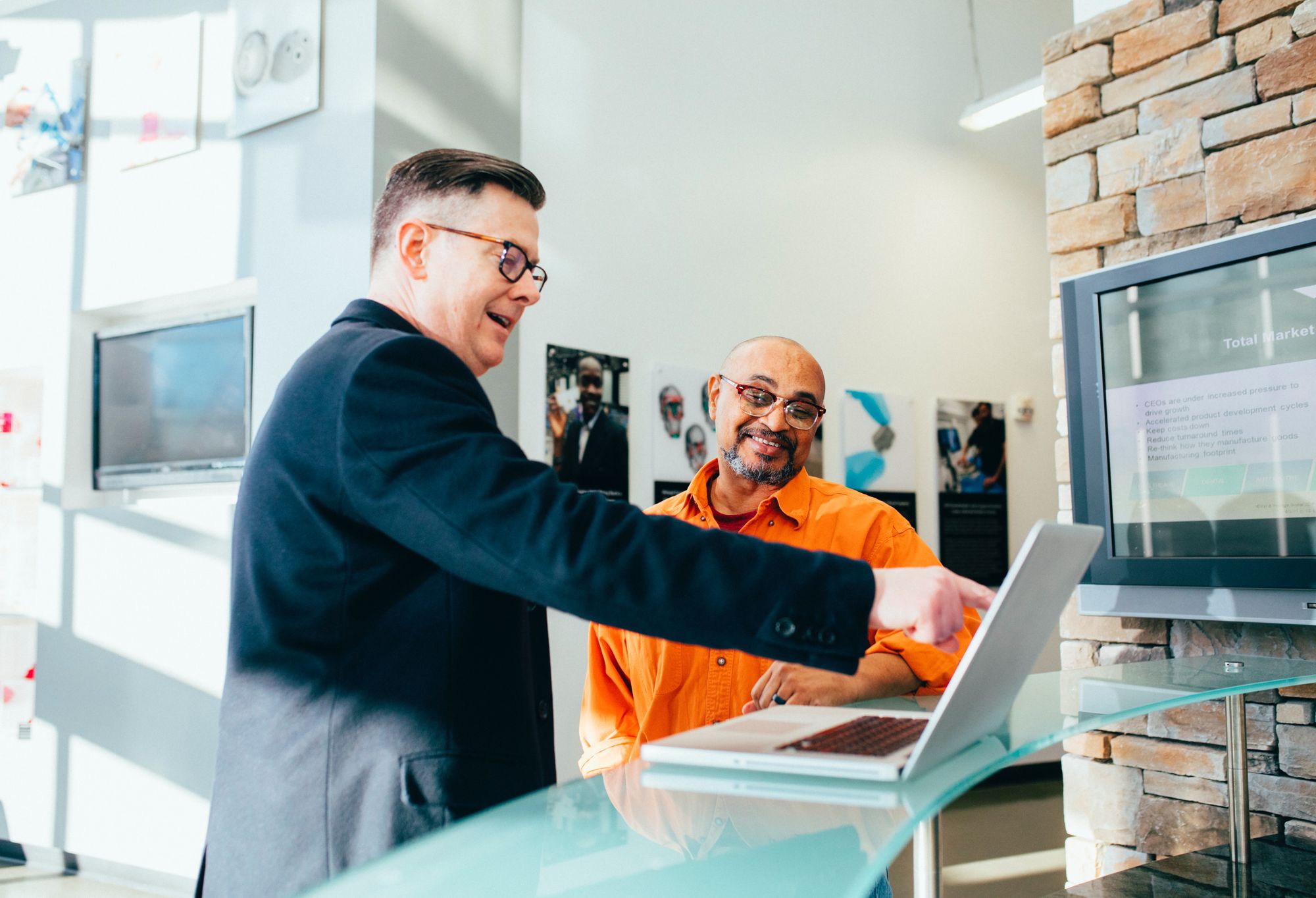
Software Recommendations
Developing a state-of-the-art digital twin necessitates the use of sophisticated software that can support complex simulations and data analysis.
The following software has proved successful in their respective industries:
- SmartSignal and Asset Performance Management by GE: provide real-time monitoring of jet engines, wind-farms, off-shore oil rigs, power generation equipment, pumps, compressors, chillers, and more.
- Tecnomatix by Siemens: a comprehensive digital manufacturing software suite designed to streamline and optimize manufacturing processes, offering the two powerful products of Process Simulate and Plant Simulation.
- aPriori Digital Manufacturing Simulation Software: cloud-based platform used by manufacturers to unlock cost, manufacturability, and carbon insights for increased product profitability and sustainability. Geared toward aerospace, defense, automotive, and industrial equipment industries.
- TeliportMe Virtual Tour Software: enables users to create immersive and interactive virtual tours using panoramic images and 360-degree photographs. Best for property documentation.
Applications of TeliportMe's virtual tour software range from insurance documentation to digital marketing, providing a versatile solution for various industries.
Data Management Considerations
The concept of a digital twin is incomplete without a robust data management strategy. Data is the lifeline that fuels the capabilities of a digital twin, providing insights and predictive analytics that empower decision-makers. In this section, we delve into the essential aspects of Data Management in Digital Twins, focusing on data quality, security, and efficient storage and access.
Data Quality and Consistency
Inaccurate or inconsistent data can lead to erroneous conclusions, potentially compromising the digital twin's effectiveness. Ensuring precise and reliable data involves:
- Implementing stringent data validation processes to verify data accuracy.
- Utilizing advanced algorithms to cleanse and normalize datasets.
- Establishing rigorous protocols for data entry and collection.
- Continuous monitoring and refining of data quality metrics.
Efficient Data Storage
The aim is to manage the volume, velocity, and variety of data generated. Effective strategies include:
- Leveraging cloud services for scalable and flexible data storage solutions.
- Utilizing state-of-the-art database management systems for quick data retrieval.
- Employing data warehousing techniques to organize and store large datasets.
- Ensuring real-time data access to keep the digital twin up-to-date with its physical counterpart.
Extrapolating Insights
With the vast amounts of data collected from IoT devices, Big Data analytics become paramount in driving actionable insights. Some strategies include:
- Implement advanced analytics and machine learning algorithms.
- Develop data visualization tools for clearer interpretation.
- Utilize predictive modeling to anticipate future trends.
- Foster collaboration between data scientists and domain experts for comprehensive insights.
Data Security and Privacy
Data security and privacy must be treated with the highest priority. Sensitive information must be safeguarded against unauthorized access and cyber threats. Key practices to enhance data security and privacy include:
- Encryption of data in transit and at rest to protect against breaches.
- Adoption of robust authentication mechanisms to ensure data integrity.
- Regular audits to identify and rectify potential security vulnerabilities.
- Compliance with global data protection regulations to preserve user privacy.
The Importance of Standardization and Interoperability
A critical aspect that often determines the success and scalability of virtual replicas is the ability to maintain standardization and interoperability. This section explores why setting universal standards is crucial and how industry-wide efforts are paving the way for interoperability.
Importance of Universal Standards
Universal standards in digital twin technologies serve as the backbone for creating robust and interoperable systems. Harmonized standards ensure that different digital twins can communicate and work together effectively, fostering an ecosystem where data and insights can be shared across platforms and industries. This not only enhances the value of digital twins but also contributes to the reduction of integration costs and complexities.
Efforts Toward Interoperability Among Diverse Systems
Interoperability is the key to unlocking the full potential of digital twins. It allows for the seamless exchange of information between various digital twins, even when they are developed by different organizations or for different purposes. This synergy is crucial for large-scale projects, such as smart city initiatives or integrated healthcare systems, where multiple digital twins must operate in concert.
The Role of Consortia and Industry Groups
Recognizing the need for a unified approach, consortia and industry groups have emerged as major players in the drive toward standardization and interoperability. These organizations work collaboratively to devise guidelines, frameworks, and protocols that facilitate the widespread adoption and integration of digital twin technologies. Through these efforts, they aim to establish a common language and shared best practices that underpin the advancement of digital twin ecosystems worldwide.
- Development of industry-specific standards and models
- Collaborative projects between companies and research institutions
- Global initiatives that define data exchange protocols
By addressing these aspects, the industry can ensure that digital twins will serve as a driving force in the era of digital transformation.
Case Studies:
Digital twins have proven their mettle across various industries, highlighted by these case studies:
- Aerospace: NASA's Digital Twins on Mars
- Manufacturing: General Electric’s Gas Turbine Power Plant
- Healthcare: Mayo Clinic’s Patient-Specific Digital Twins
- Energy: Siemens’ Virtual Power Plant
- Construction: Cityzenith’s Digital Twin Platform
- Transportation: Singapore Land Transport Authority's Digital Twin for Intelligent Transportation Systems
- Retail: Walmart’s Digital Twin for Supply Chain Optimization
In each instance, the digital twin served as a robust tool for pre-empting real-world challenges, heralding a new era of predictive analysis and strategic foresight.
Let's look at these cases in further detail:
Aerospace: NASA's Digital Twins on Mars
Perseverance, the Mars rover on a mission to explore the Jezero crater, was launched on July 30, 2020, and successfully landed on Mars on February 18, 2021. As one of NASA's most advanced rovers at the time, it marked a significant step in space exploration. The entire landing process, despite being a complex design involving intricate technology like the sky crane, went off without a hitch.
Digital twin technology played a crucial role in making this mission a success. In the early stages of Perseverance's development, NASA extensively used detailed virtual models to anticipate and plan for the challenges the rover would face on Mars. The digital twin simulations also included the sky crane technology, ensuring a safe and flawless landing on the Martian surface.
To further enhance the rover's capabilities, NASA employed MapleSim by Maplesoft for hardware-in-the-loop simulations. This approach allowed them to simulate specific hardware components alongside digital twins, ensuring a comprehensive understanding of the rover's functions. Additionally, a research team from McGill University and Vortex simulated how the rover's wheels would interact with soft patches on Mars, contributing valuable insights.
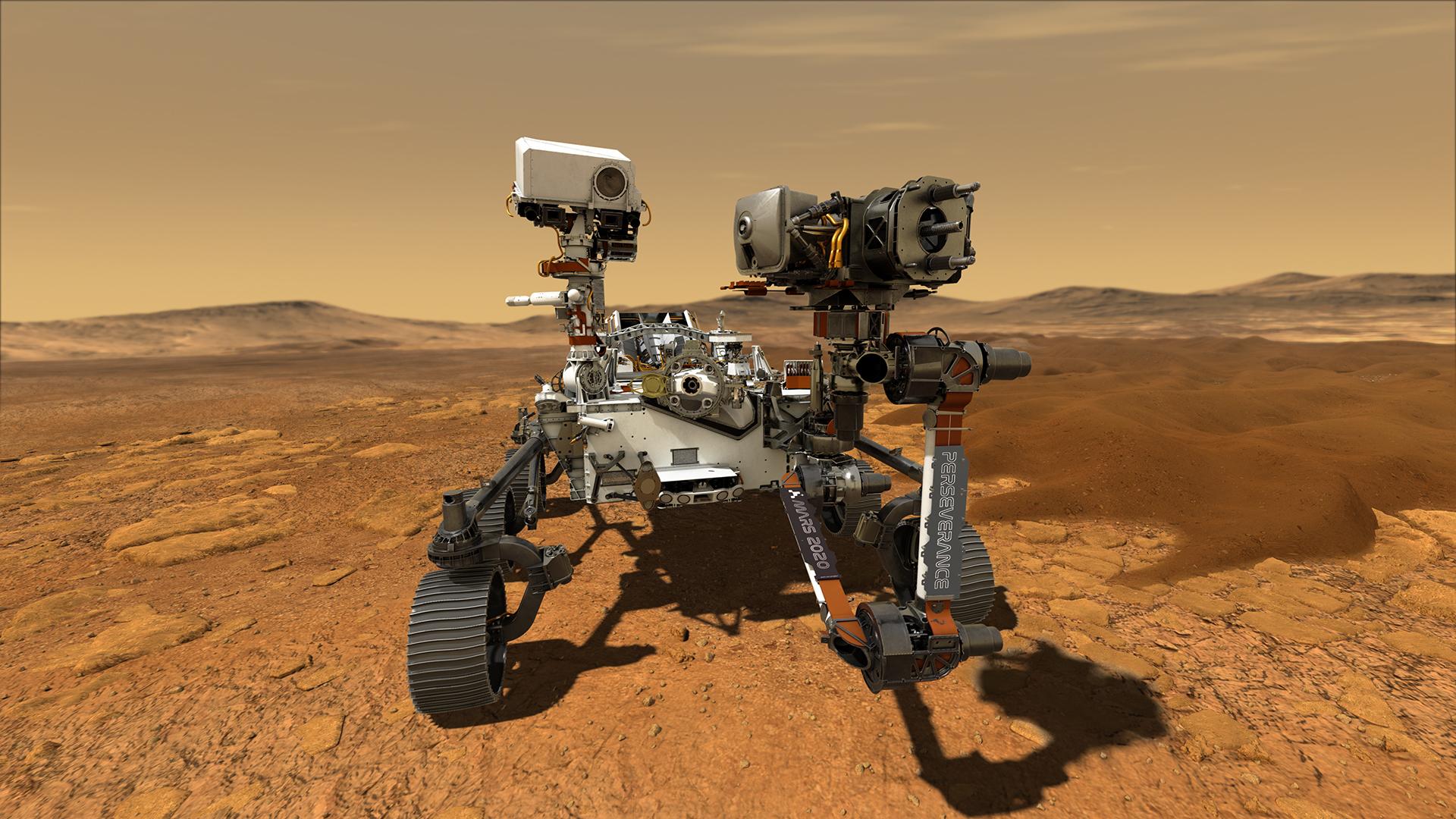
.jpg)
Manufacturing: General Electric’s Gas Turbine Power Plant
General Electric’s Gas Turbine Power Plant in Bouchain, France, recently set an efficiency record by turning 62% of its fuel's energy into electricity. To achieve this result, a digital twin system gathers real-time data from sensors installed in the turbines, analyses it, and offers suggestions for optimal performance.
In comparison to older plants with around 3,000 sensors, newer ones like Bouchain have 5,000 to 6,000 sensors which give the plant a constant MRI. This digitization not only detects tiny changes that might signal a need for maintenance but also significantly reduces the chance of unexpected or risky surprises.
Beyond just the turbine, this digital approach extends to the entire power plant, guiding operations through analysis, data, and personalized solutions.
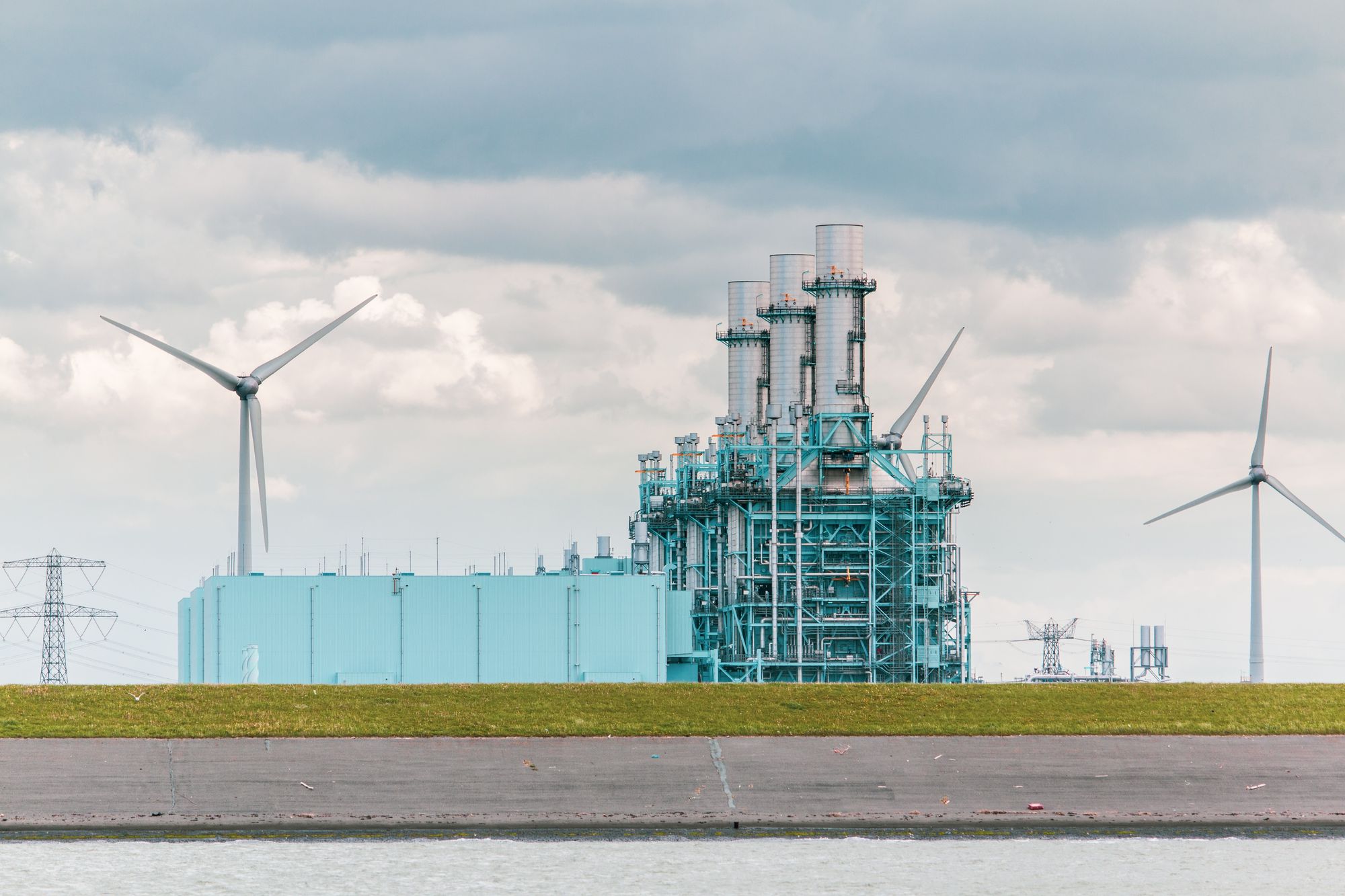
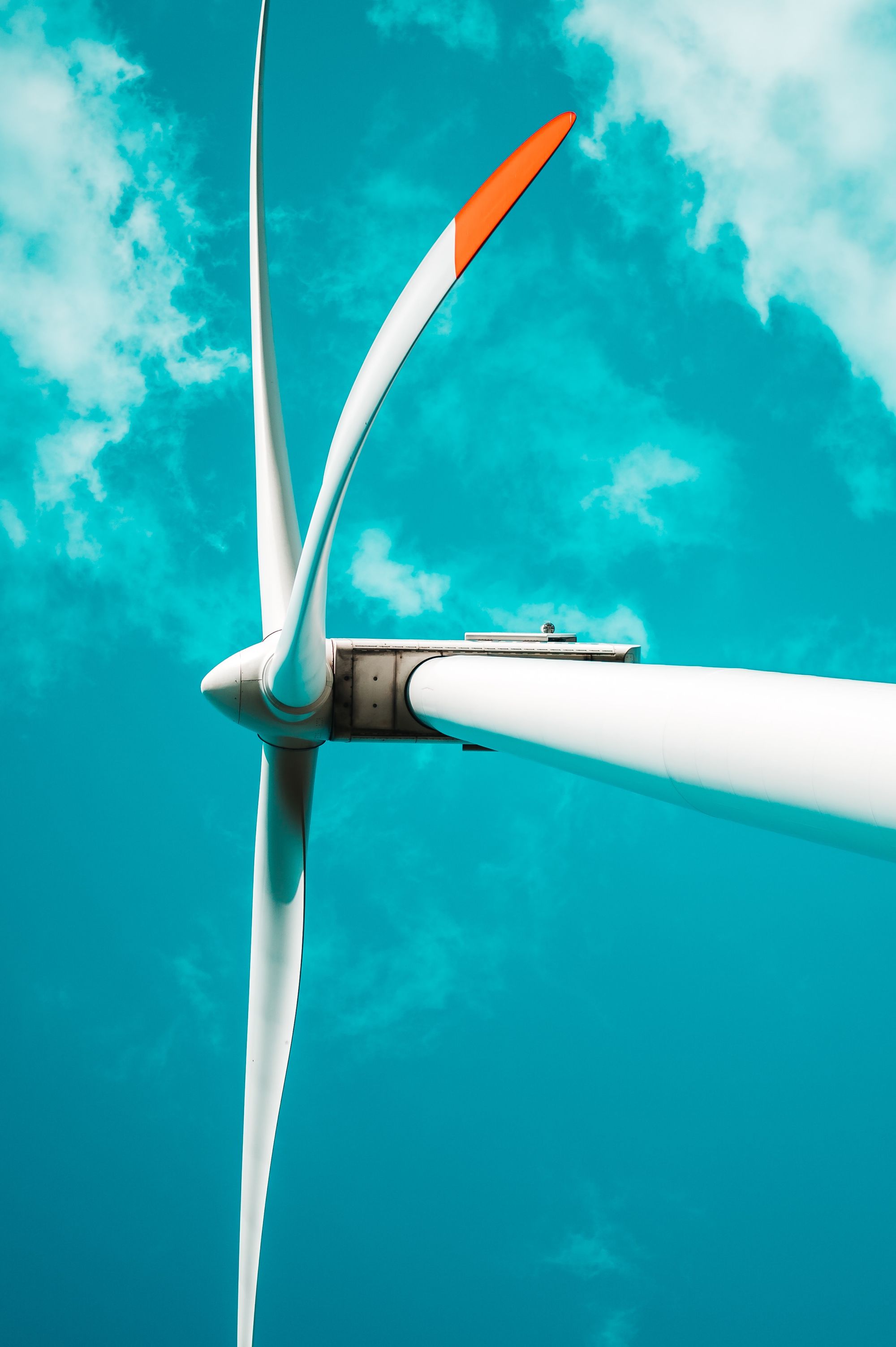
Healthcare: Mayo Clinic’s Patient-Specific Digital Twins
The Mayo Clinic, a renowned healthcare provider, has leveraged digital twin technology to create patient-specific models for improved diagnostics and treatment. By integrating data from various sources such as medical imaging, genetic information, and wearable devices, the Mayo Clinic develops personalized digital twins of patients.
These digital twins simulate the patient’s physiology, enabling physicians to gain a deeper understanding of their condition and design targeted treatment plans. By analyzing the digital twin, doctors can explore different treatment options, predict the effectiveness of interventions, and optimize patient outcomes.
This approach has been particularly effective in areas like cardiac care, where the digital twin helps in planning complex surgeries and predicting the impact of interventions on individual patients.
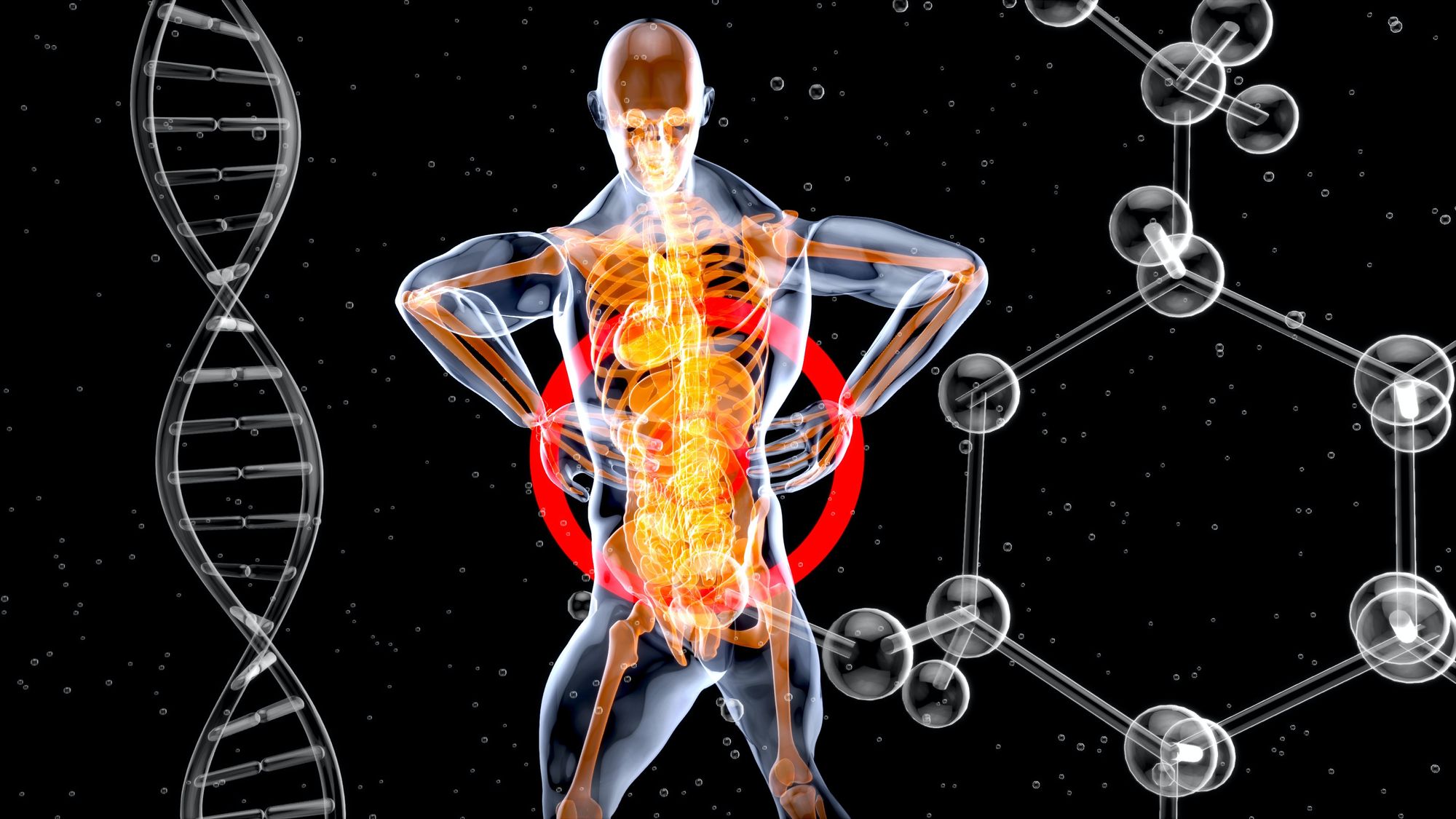
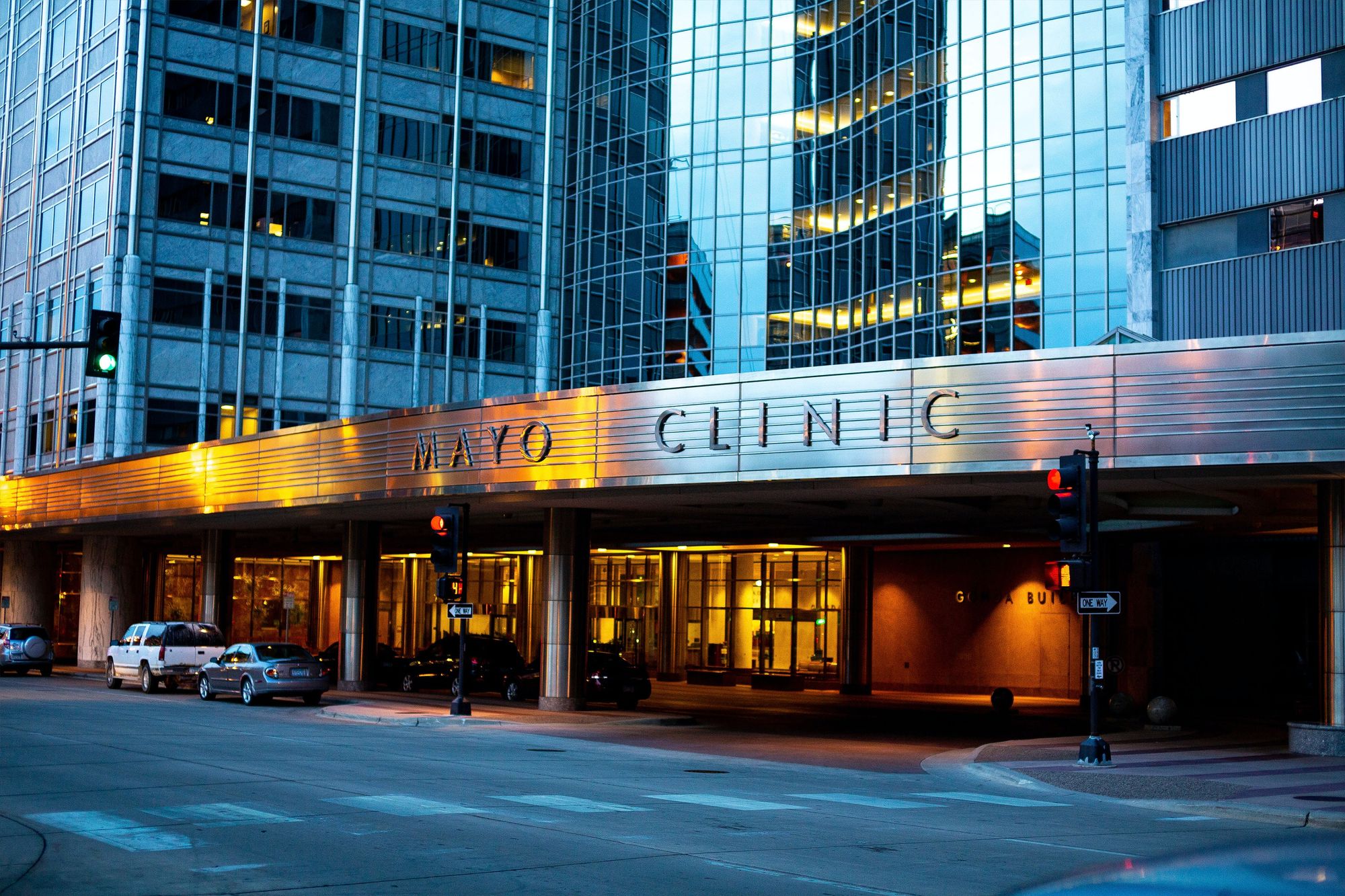
Construction: Cityzenith’s Digital Twin Platform
A full digital twin platform called SmartWorldPro has been created by Cityzenith, a technology business that specializes in urban planning and development. The platform builds digital twins of cities using data from a variety of sources, including 3D city models, BIM (Building Information Modelling) data, and IoT devices.
The digital twin enables developers and city planners to simulate various development scenarios, optimize resource allocation, visualize complex urban settings, and analyze various aspects of infrastructure.
Additionally, it promotes stakeholder participation, empowering them to speak and act with clarity. Urban planning initiatives all over the world have benefited greatly from the application of Cityzenith’s digital twin platform, making cities more resilient, sustainable, and citizen-focused.
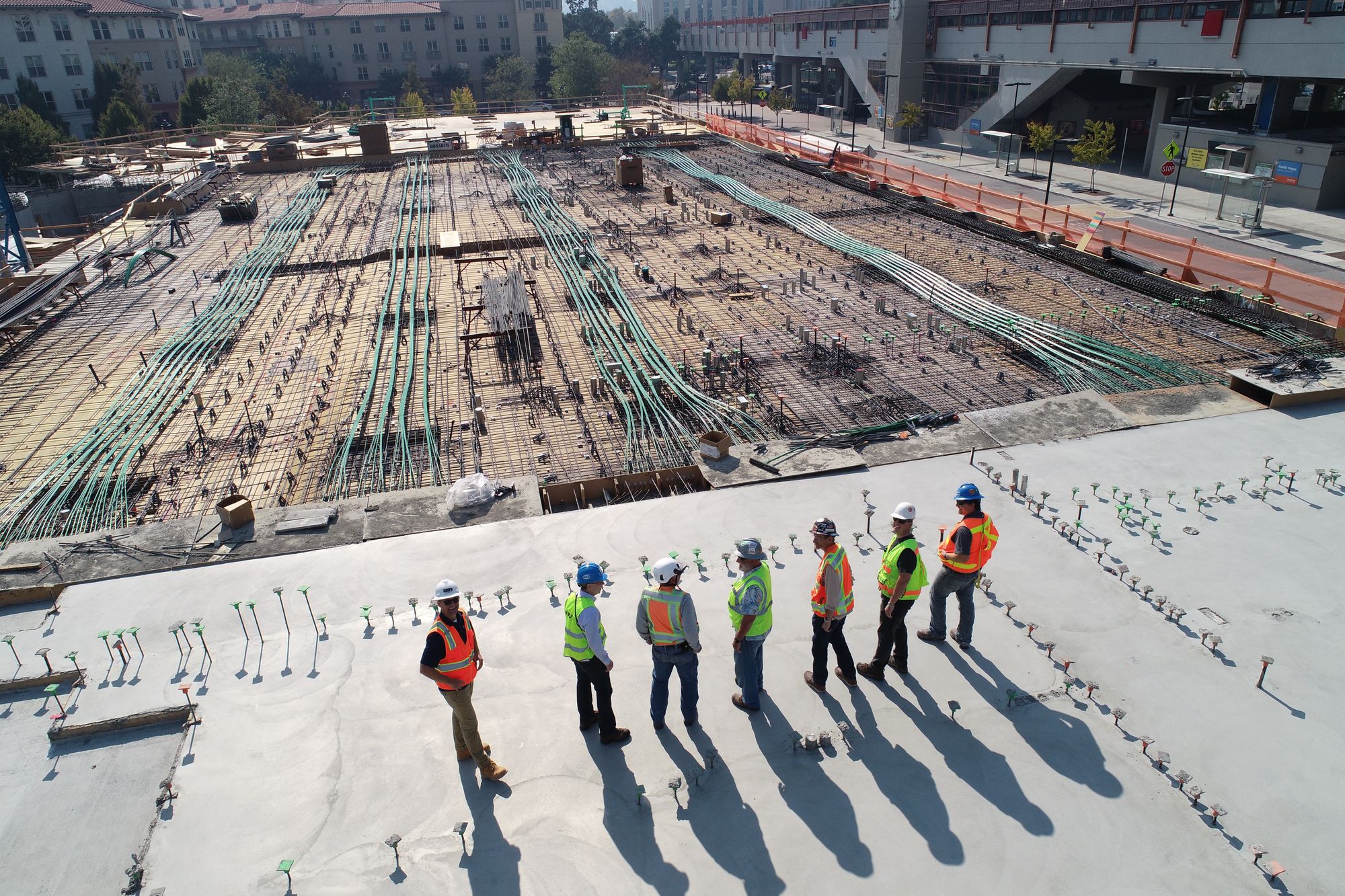
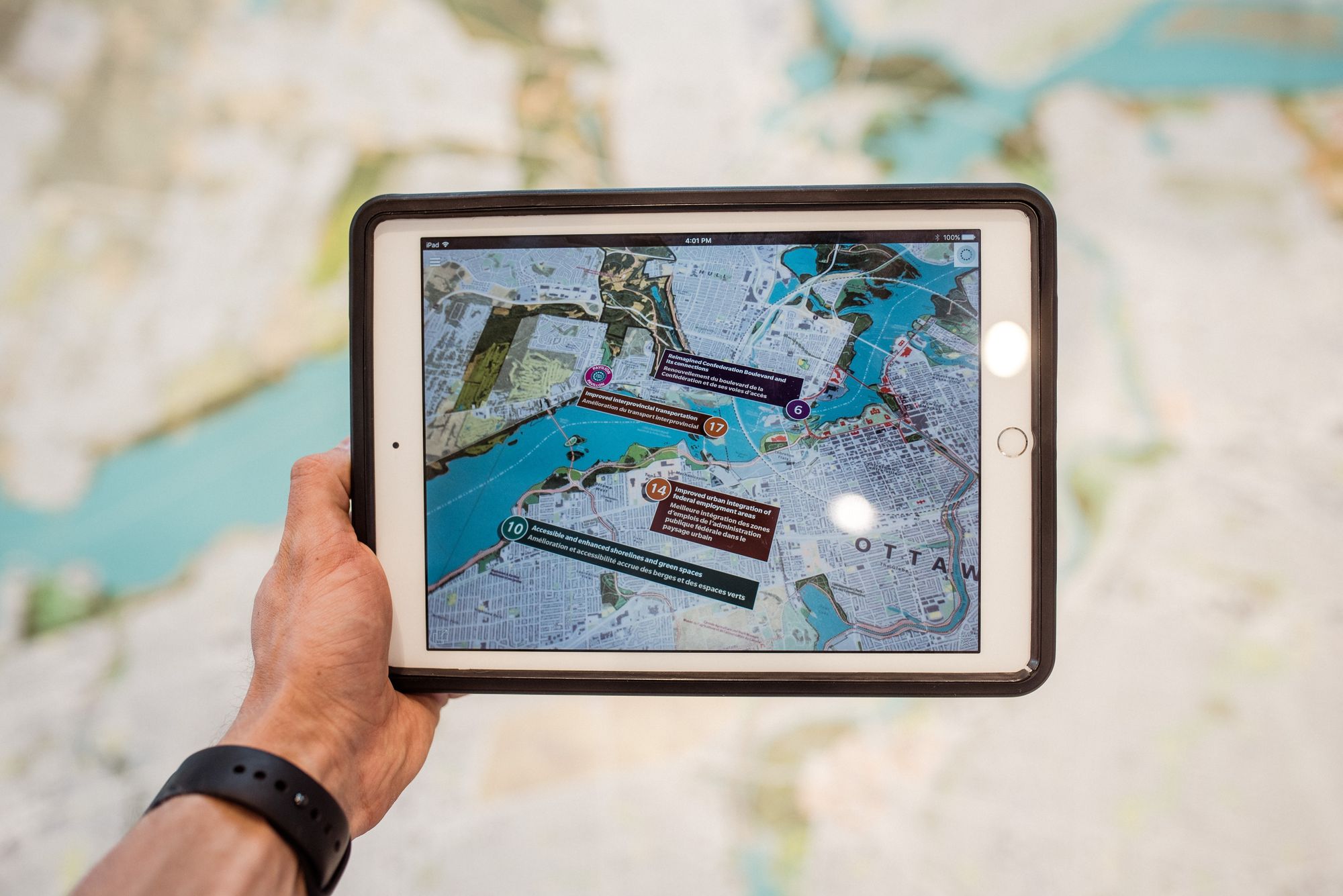
Transportation: Digital Twin for Singapore Land Transport Authority
The adoption of digital twin technology by the transport sector aims to increase the effectiveness and security of transport networks. One such instance is the digital twin of the complete transport system, including the roads, trains, and bus services, that the Singapore Land Transport Authority (LTA) has put in place. To give a complete picture of the transportation ecosystem, the digital twin incorporates real-time data from traffic sensors, fare collection systems, and other sources.
The LTA’s digital twin offers real-time traffic monitoring, enabling authorities to control congestion, optimize traffic flow, and increase overall transportation effectiveness. The digital twin aids in the planning and execution of transport infrastructure enhancements, such as the construction of new roads or public transit lines, by modeling various scenarios.
Additionally, it supports predictive analytics for incident management, enabling early issue discovery and effective interruption response.
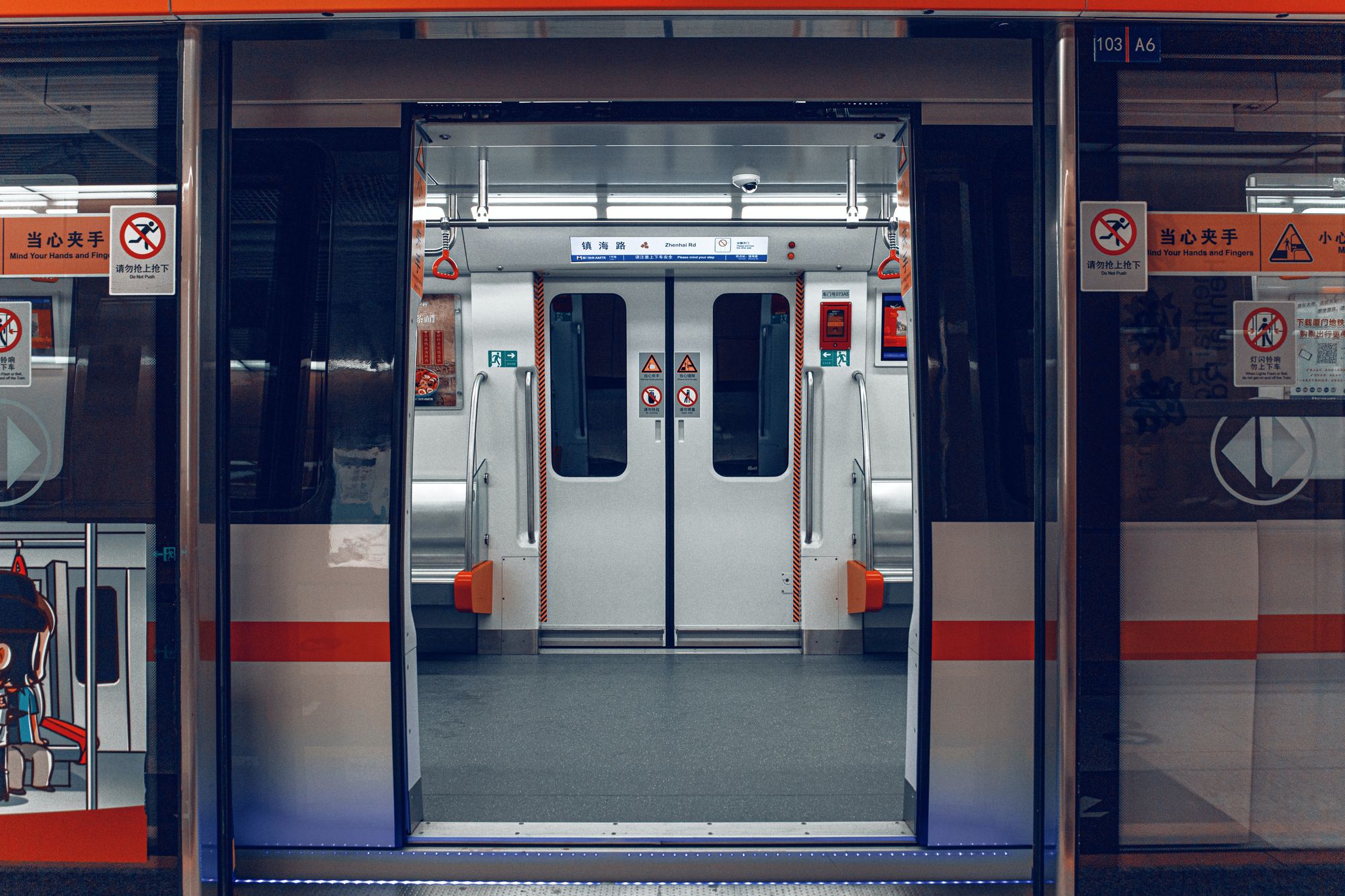
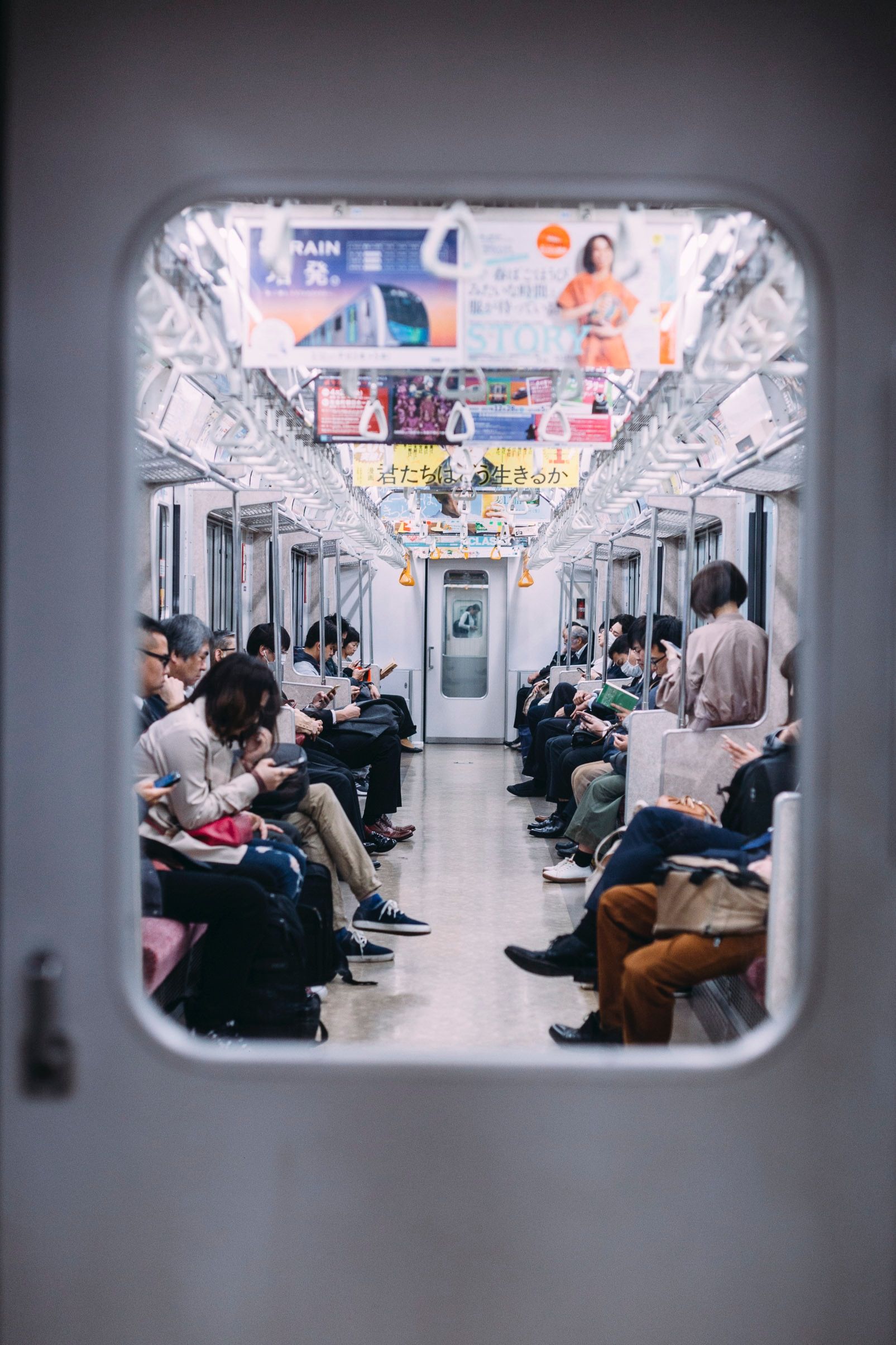
Retail: Walmart’s Digital Twin for Supply Chain Optimization
To improve its supply chain processes, Walmart, a global retail organisation, deployed a digital twin solution. To simulate and analyse the complete supply chain network, the digital twin integrates real-time data from numerous sources, such as sales data, inventory levels, and weather forecasts.
Walmart can correctly estimate demand, optimise inventory levels, and enhance logistics and distribution procedures by using the digital twin. Additionally, the digital twin enables simulations and scenario planning to locate possible bottlenecks and enhance the flow of commodities. Customer satisfaction has increased as a result of this application, which has improved inventory management and decreased waste.
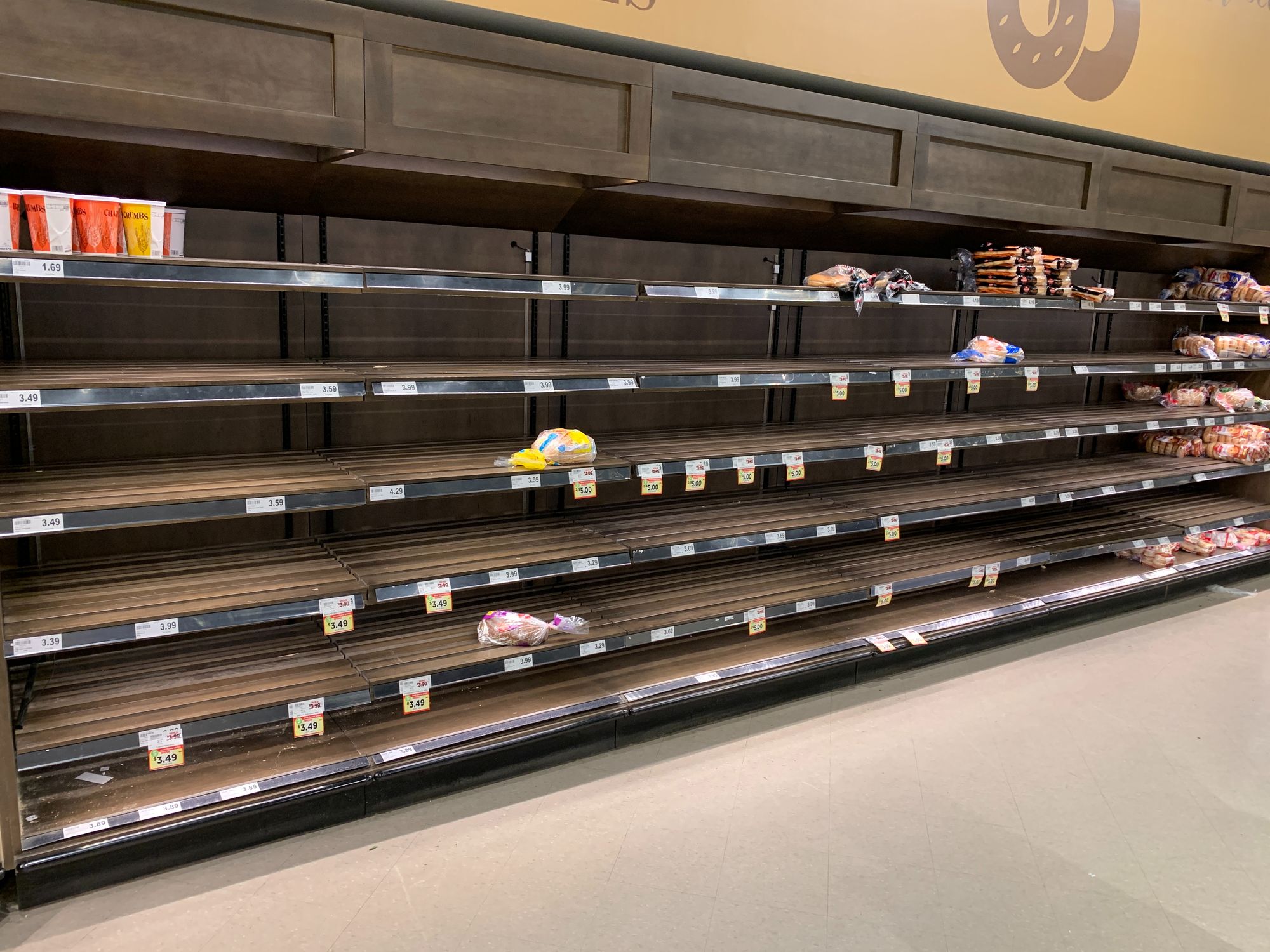
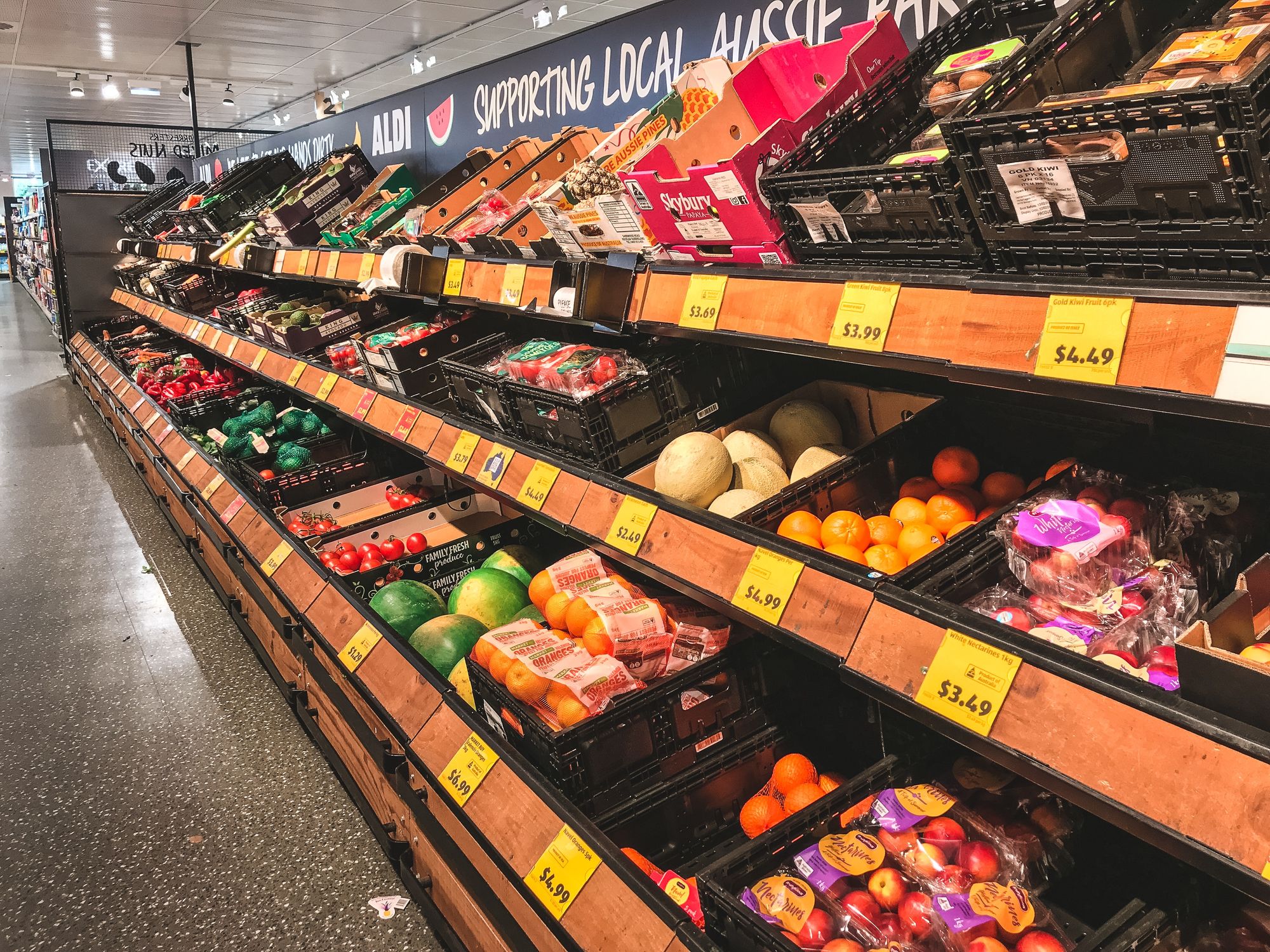
Integrating Digital Twins with AR/VR
Integrating digital twin technology with augmented reality (AR) and virtual reality (VR) enhances visualization and provides innovative ways for training and prototyping.
Enhancing Visualization and Interaction with Models
By overlaying digital information onto the physical realm (AR) or creating a fully immersive simulation environment (VR), engineers and stakeholders can interact with their digital twins in a more intuitive and meaningful way. This leads to better understanding of the data, quicker decision-making processes, and a more holistic view of the operational aspects of the twin.
Training and Prototyping Using AR/VR
Trainees can practice operations on virtual models that react in real-time, reducing the risks and costs associated with hands-on training. With regard to prototyping, designers and engineers can test and iterate their models in a virtual environment before any physical prototype is built, saving time and resources while accelerating innovation.
Future Outlook of Digital Twin Integration with AR/VR
The future of digital twins is poised to be deeply intertwined with immersive technologies. As the resolution of VR and AR continues to increase and as the hardware becomes more accessible, the line between physical and digital will blur. This promises a new era where digital twin immersion becomes a staple across industries, providing an unparalleled level of detail and interactivity that will drive the next wave of digital transformation.
- Analyzing complex systems through a more interactive lens
- Reducing gaps in understanding by providing a tangible reference through AR overlays
- Advancing remote collaboration through shared VR environments
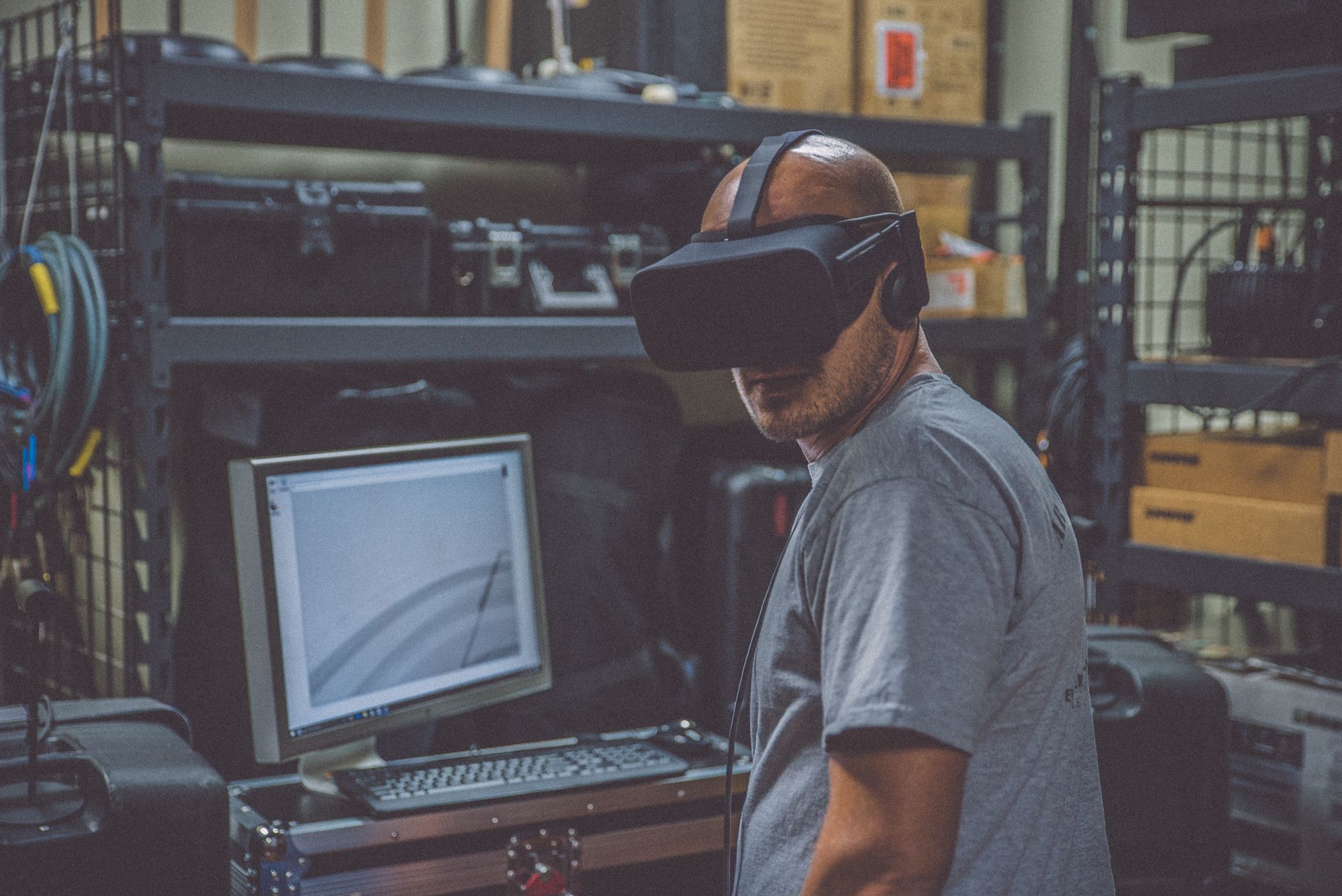
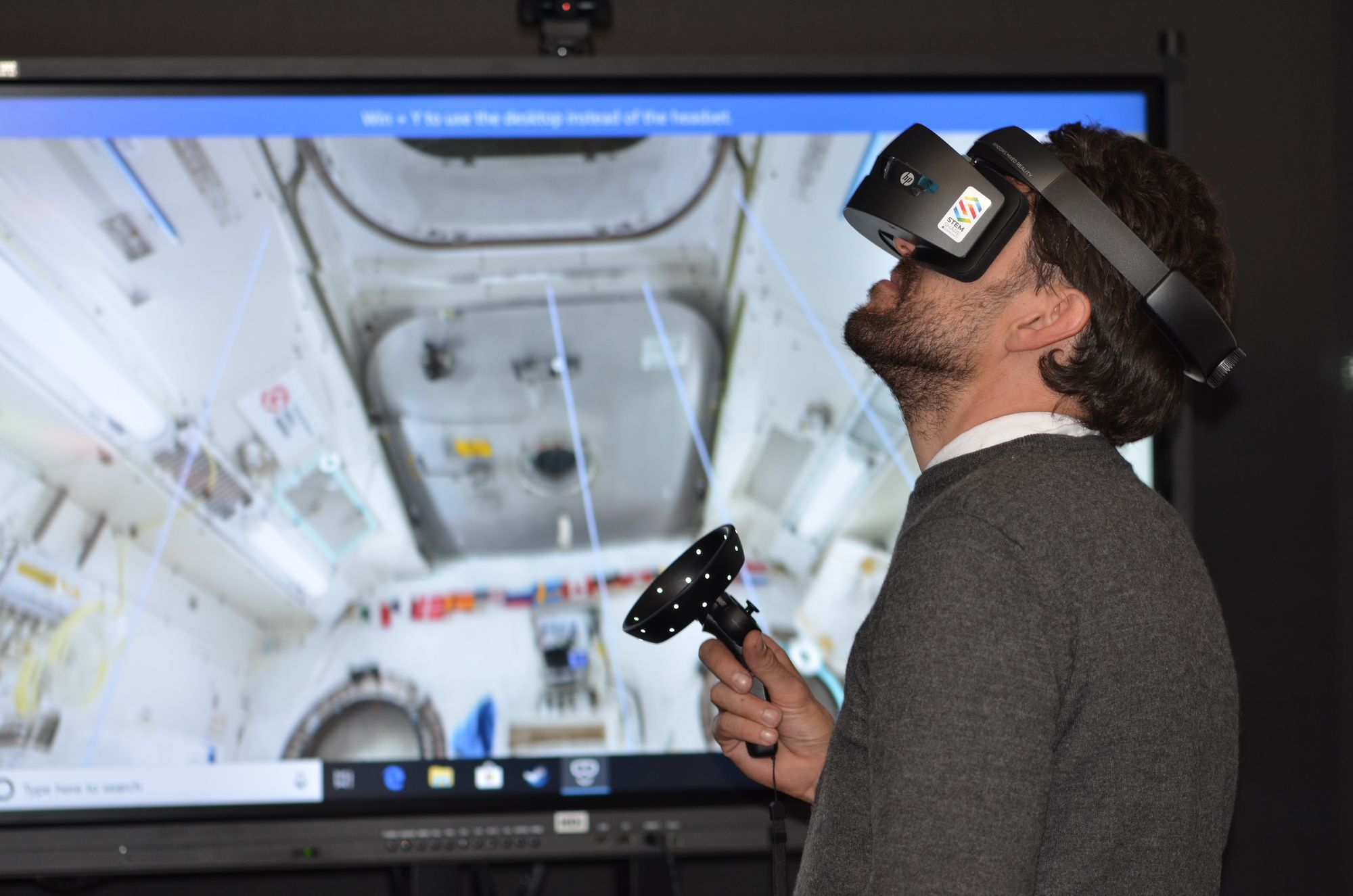
Cybersecurity Considerations
As the digital and physical worlds merge closer, securing digital twin data becomes a paramount concern. The interconnectedness poses risks of unauthorized access, manipulation, or exploitation of sensitive information, emphasizing the need for robust cybersecurity measures to safeguard the integrity, confidentiality, and privacy of digital twin data.
As a result, it's critical to understand and implement rigorous cybersecurity measures to protect sensitive data and the systems they mirror and manage.
We suggest the following practices to safeguard your data:
1. Secure Data Across Lifecycle Phases
Data is the lifeblood of digital twins, accompanying assets from inception to retirement. Each stage of the digital twin's lifecycle poses potential vulnerabilities that must be addressed:
- Creation: During this phase, ensuring that the initial data is accurate and comes from reliable sources is crucial for the twin's effectiveness and security.
- Operation: Real-time data streams require encryption and vigilant monitoring to avoid breaches or tampering.
- Maintenance: Regular updates and patches fortify digital twins against emerging threats.
- Decommissioning: Properly disposing of data in the twin is just as important, preventing unauthorized access to historical data.
2. Analyze Risk
A methodical approach to identifying and mitigating potential threats, threat modeling, is vital in the lifecycle of a digital twin. By understanding the various ways a digital twin can be compromised, organizations can implement tailored defenses. This anticipatory measure involves:
- Analyzing the attack surfa ce of the digital twin environment.
- Identifying potential threat agents and the potential impact of their actions.
- Developing a priority list of risks to address based on their severity and likelihood.
3. Establish Robust Security Practices
Robust security tactics are non-negotiable when it comes to digital twin implementations. To safeguard these complex systems, consider the following:
- Multi-Factor Authentication (MFA): Requiring more than one method of authentication enhances access security.
- Encryption: Protecting data in transit and at rest ensures that even if intercepted, the information remains unreadable.
- Segmentation: Limit potential damage by compartmentalizing networks and systems.
- Zero Trust Architecture: Never assume security within the network; instead, verify every access request as if it originates from an open network.
- Continuous Monitoring: Implement real-time security monitoring to detect and respond to threats swiftly.
- Regular Audits: Conduct security audits to uncover any potential weaknesses and ensure compliance with industry standards.
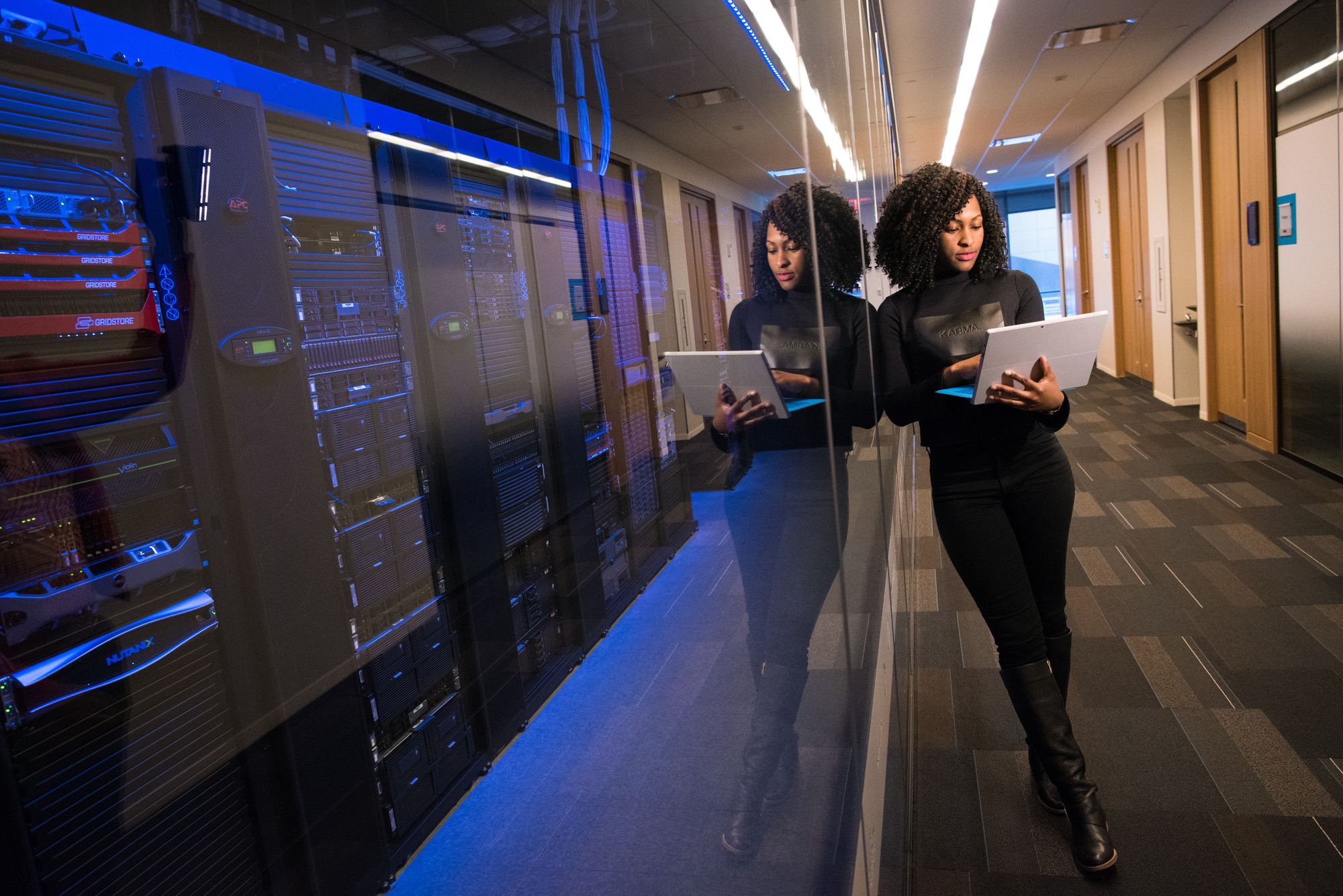
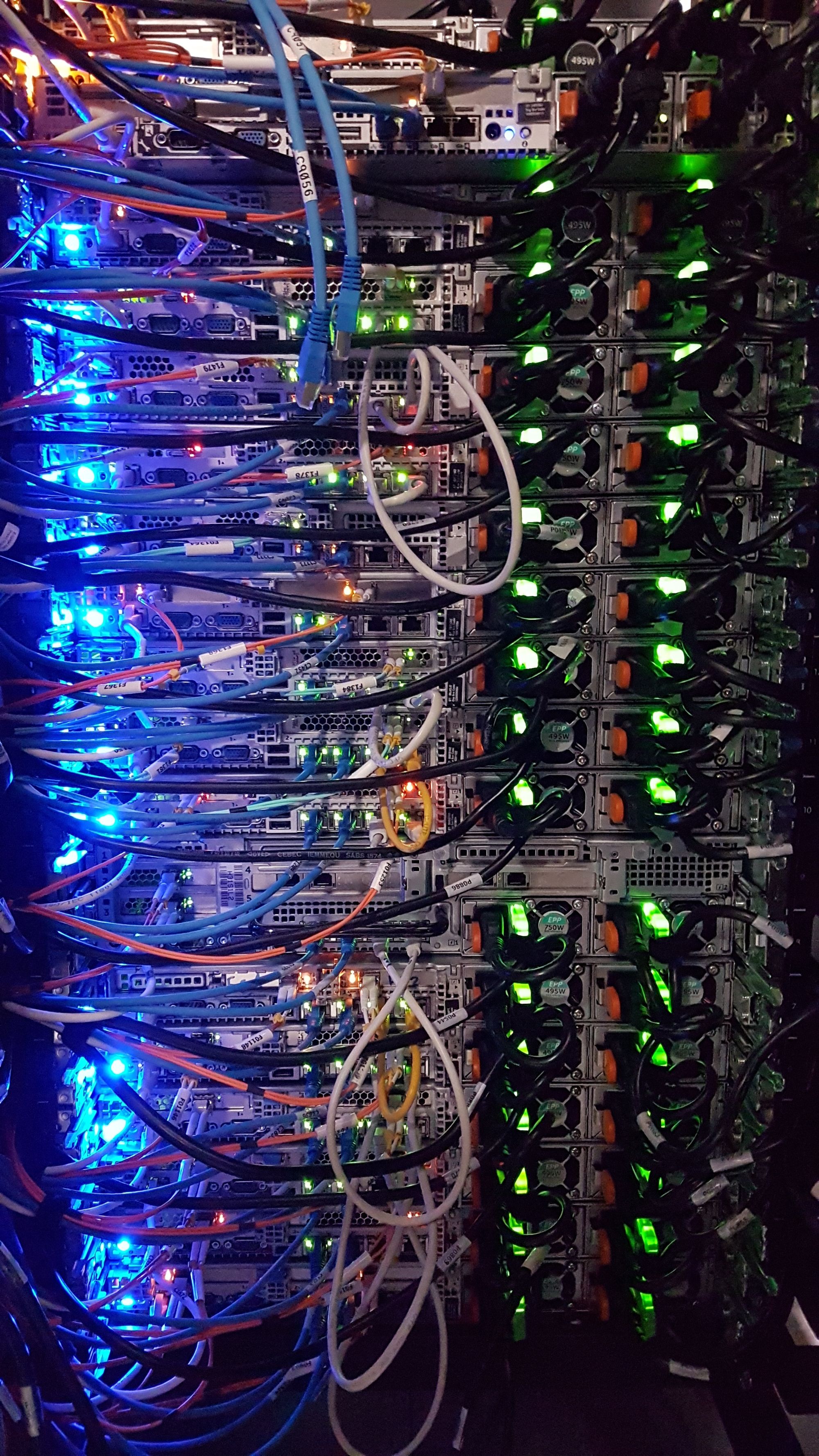
Future Predictions for Digital Twin Technology
Digital twin technology is on the cusp of a transformative journey, poised to redefine how industries operate and innovate. As we peer into the future, several key predictions emerge
- Increased Adoption Across Industries: Digital twin technology is likely to experience widespread adoption across various industries beyond its current applications in manufacturing, healthcare, and energy. Sectors such as logistics, construction, and smart cities are expected to embrace digital twins to enhance efficiency, decision-making, and overall operations.
- Advancements in AI Integration: The integration of artificial intelligence (AI) with digital twin technology is anticipated to grow, enabling more sophisticated data analysis and predictive capabilities. AI algorithms will play a crucial role in extracting actionable insights from the vast amount of data generated by digital twins, further enhancing their value.
- IoT Integration for Real-time Monitoring: The Internet of Things (IoT) will play a more integral role in digital twin ecosystems, enabling real-time monitoring and control of physical assets. This integration will enhance the accuracy and immediacy of data, providing a more dynamic and responsive digital representation of the physical world.
- Expansion into Edge Computing: As edge computing capabilities evolve, digital twin processing is likely to shift towards the edge of networks. This move will reduce latency, enhance real-time decision-making, and enable more efficient use of resources, especially in scenarios where immediate response is critical.
- Standardization and Interoperability: The development of industry standards and increased interoperability between different digital twin platforms is expected. This will facilitate seamless collaboration, data sharing, and integration across various systems and domains, fostering a more connected and efficient ecosystem.
- Digital Twins in Healthcare: The healthcare industry is poised to see increased adoption of digital twins for personalized medicine, patient monitoring, and simulation of medical procedures. This technology could revolutionize healthcare practices by providing more accurate and individualized insights.
- Enhanced Cybersecurity Measures: Recognizing the increasing importance of securing digital twin data, there will be a concerted effort to implement robust cybersecurity measures. This will include encryption, access controls, and other security protocols to protect sensitive information associated with digital twins.
- Human-Machine Collaboration: Future digital twins may facilitate more seamless collaboration between humans and machines. This could involve advanced augmented reality interfaces, enabling workers to interact with and manipulate digital twins in real-time for tasks ranging from maintenance to design.
- Lifecycle Integration: Digital twins are likely to become more integral throughout the lifecycle of products, assets, or systems. From design and development to operation and maintenance, a continuous and evolving digital representation will provide valuable insights and support decision-making at every stage.
- Environmental Sustainability: Digital twins may play a key role in promoting environmental sustainability by optimizing resource usage, energy efficiency, and waste reduction. Industries and cities may leverage digital twins to model and implement eco-friendly practices and strategies.
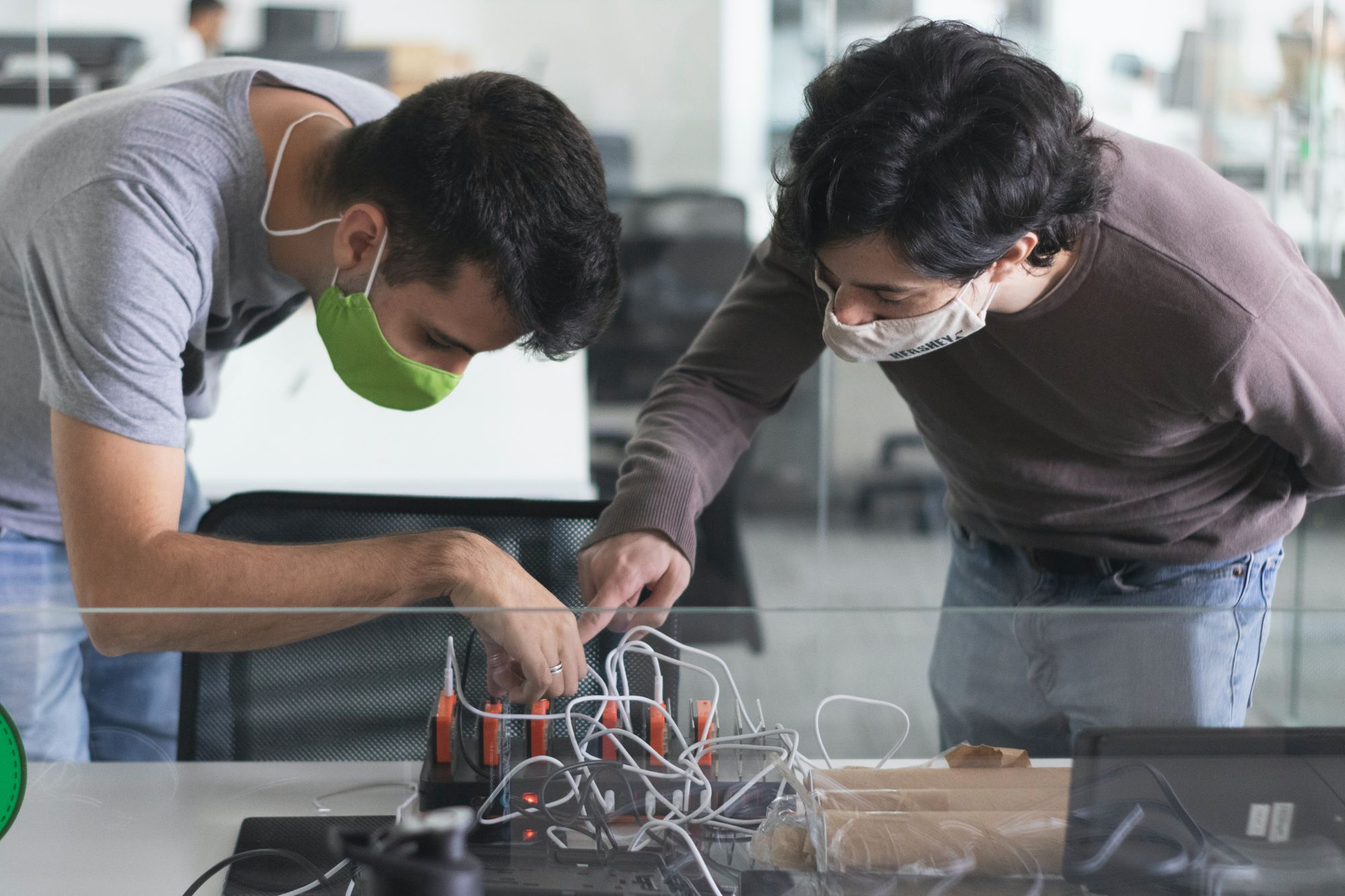
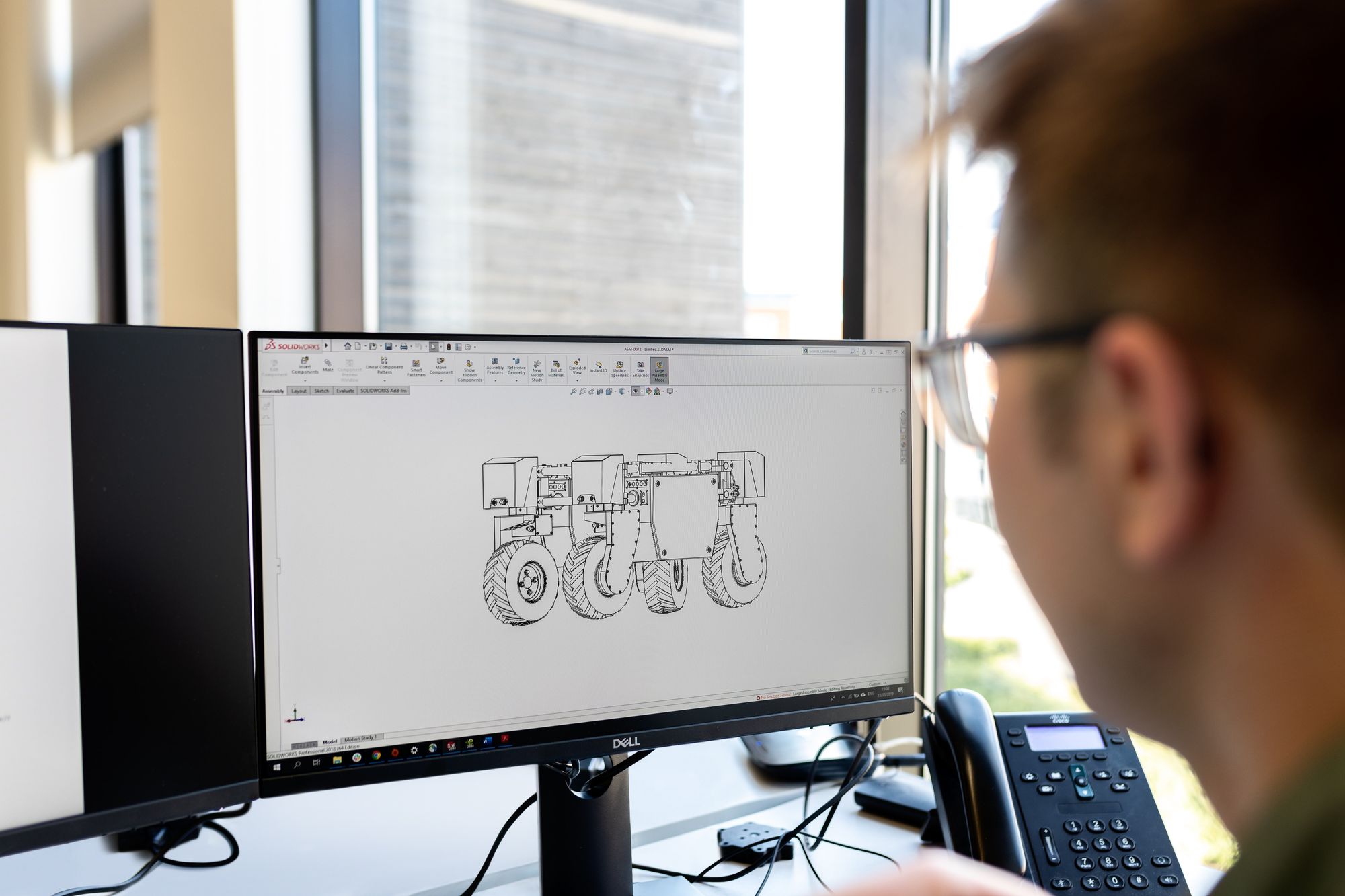
Key Takeaways and Steps Forward
- Digital twins optimize processes, streamline operations, and improve overall efficiency across various industries
- By simulating scenarios and analyzing data, digital twins empower better decision-making, particularly in complex systems
- Digital twins have transformative potential across sectors, contributing to advancements in technology, sustainability, and innovation.
- In taking steps forward, emphasis must be placed on overcoming challenges related to scalability, integration, and standardization.
- As these virtual constructs become even more sophisticated and integral to our digital infrastructure, the focus must also be on nurturing the skills and knowledge base required to leverage their full potential.